DATA GOVERNANCE
Key Considerations in Establishing Sustainable Data Governance
By Betty Ann M. Turpin, Ph.D
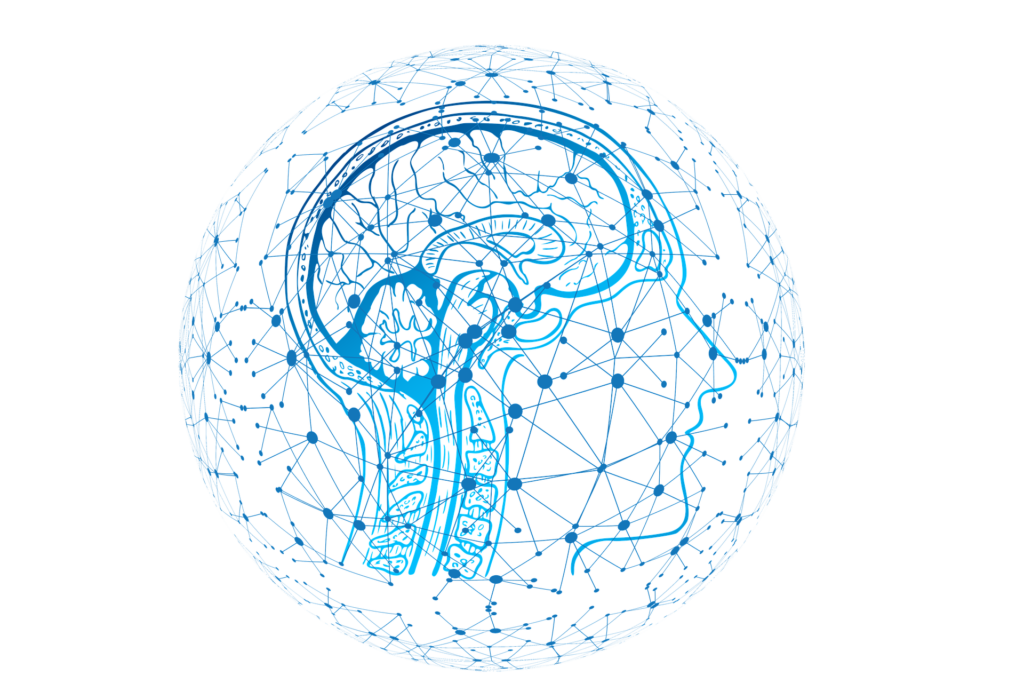
Organizations are in a constant state of flux, even with a 3-5 year strategic plan “in-hand”. Today, constant changes are driven not only by external factors, but by the greater reliance on information management and technology systems (IM/IT). With data at the heart of many government digital transformation initiatives, sustainable data governance is paramount. This article explores how data governance practices and key lessons can help to address this challenge in a continually changing environment. In strictest terms, governance entails authority, decision-making, and accountability.[1] The definitions of data governance are broader and several exist. Some are simple:
- a collection of practices and processes that can support formal management of data assets;
- others are more comprehensive including data quality, stewardship, security and privacy, usability, integration, compliance, roles, integrity, tools and skills.
Inclusive data governance frameworks
Regardless of the complexity of the data governance framework adopted, a shift is needed from the former top-down, IT focused and workflow-driven [2] approaches to more eclectic and inclusive frameworks such as the SAS best practice model3, which is a comprehensive approach to data governance sustainability. The attraction of the SAS model is its comprehensiveness as that integrates several aspects to enable sustainability. Figure 1 incorporates the main elements of the SAS approach, as well as a few other considerations.
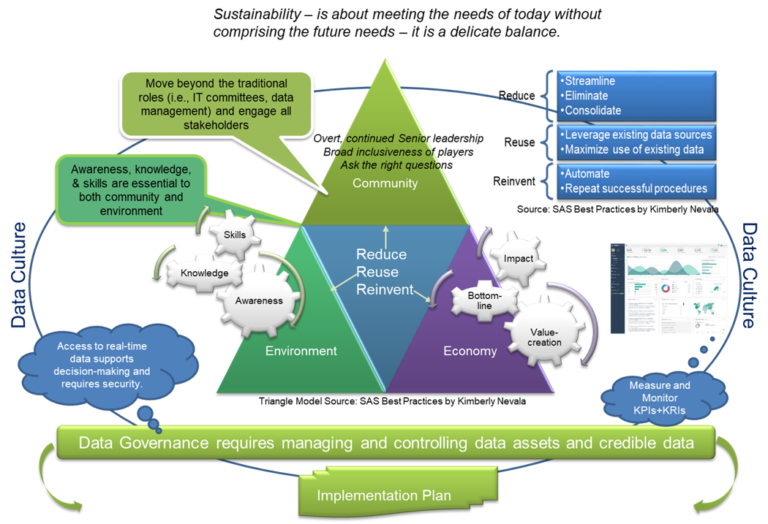
The SAS model is comprised of three primary dimensions:
Community – an inclusive body of individuals at various levels and functions, whose interest is data. Ensure that all business areas are accountable for and share responsibility for success (or failure). Create transparent awareness and knowledge about data at all levels in the organization that helps to manage expectations.
Environment – invests in and develops the knowledge, skills, and tools to manage data as an asset. Defining roles, responsibilities, and authorities for data. Governance has a long-term role in the strategic decision-making in the organization.
Economy – is about incorporating value creation, which means relying on data governance as a means to the strategic goals of the organization. Measurement is essential.
The SAS model goes beyond simply addressing IT management and assets. Each dimension calls for defining bodies and approaches for ensuring authority, decision-making and accountability. These SAS primary dimensions have been identified, in general terms, in various government data analytic strategies and/or, in part, in data culture or data literacy plans and renewed governance committees. In some cases, the dimensions are found in separate plans, which raises the concern about fragmentation, despite consultation efforts, because integration of all dimensions within the organization is necessary for sustainability.
How to apply frameworks effectively
The concept of sustainability necessitates that the framework be evergreen to adapt to ongoing changes in the organization, a necessity for sustainability success. A few key lessons have been learned in applying these types of frameworks.
First, within the community dimension, it is essential to have visible, active and ongoing senior management commitment. A major role for senior management is to manage competing priorities and increasing demands from central agencies, which often occur with little to no claw back on previous expectations.The priority focus must shift to data governance as the single most important priority, second only to the people (employees and citizens).
Second, experience has shown that committees/governing bodies create typical terms of reference (i.e., mission, roles, standards, members, model, policies/procedures) but often the goals are too lofty or vague, entailing too many levels of approval to be adaptable and timely; and exclude the functional experts.
Third, many strategies/plans or governing body terms of reference do not follow-up with an implementation plan. This oversight can lead to the plans and/or the data governance body becoming static or relying on a sub-working groups who are not resourced or empowered to effect change. As well, most data governance plans usually only address the IT asset needs. With an implementation plan, the organization will be able to position data governance as a long-term initiative ingrained into the fabric of the organization’s business.
Finally, a plan is not worth much if committed resources are not attached. The tendency in government is to do more with less, do it on the ‘side of the desk’, or if we can get to it. This will not suffice. “Reuse, reduce, and reinvent” are part of the SAS model. Resource efficiency can also be achieved with streamlining business processes and extraneous decision-making steps4. As well, the re-allocation of resources is a logical solution while ensuring that organizational strategic outcomes are not compromised. It is also crucial that management ensure that sufficient long-term resources are available to finance all roles and functions required for robust, ongoing data governance.
What can influence data governance sustainability
The foregoing arguments suggest that the data governance committee must explicitly define the right questions and identify key issues that will guide a data governance implementation plan, one that balances addressing present versus future needs. Refocusing a mindset from a singular focus on the IT system to the process of data governance is an important orientation for successful governance.
Data governance sustainability requires that the organization sees data as a strategic asset and that data governance supports the organization’s strategic outcomes. For this to happen, key performance indicators (KPIs) for the governance outcomes should be identified, as well as key risk indicators (KRIs) for both the governance outcomes and the operational implementation and management of the data governance.
Above all, two overriding factors will influence data governance sustainability. First, credibility and timeliness of the data. Otherwise, the utility of data governance is called into question, and generates frustration and disillusionment in the organization. Second, the development of a data-driven culture must absolutely have all your people onboard, aware and/or skilled (data savvy), and engaged.
About The Author
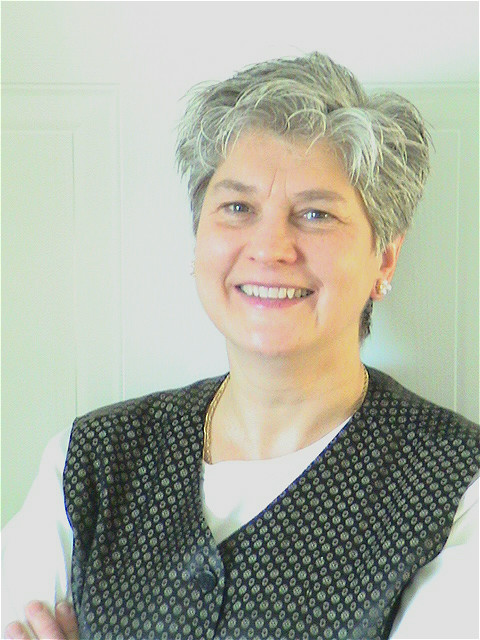
Betty Ann M. Turpin, Ph.D
President of Turpin Consultants Inc., is a management consultant who has also worked in the federal government, in healthcare institutions, and as a university lecturer. Her career focus is performance measurement, data analytics, evaluation, and research. She is a certified evaluator and coach.