FEATURES
AI Integration Helps Build the Next Generation of E-Government
By Stéphane Gagnon, Ph.D.
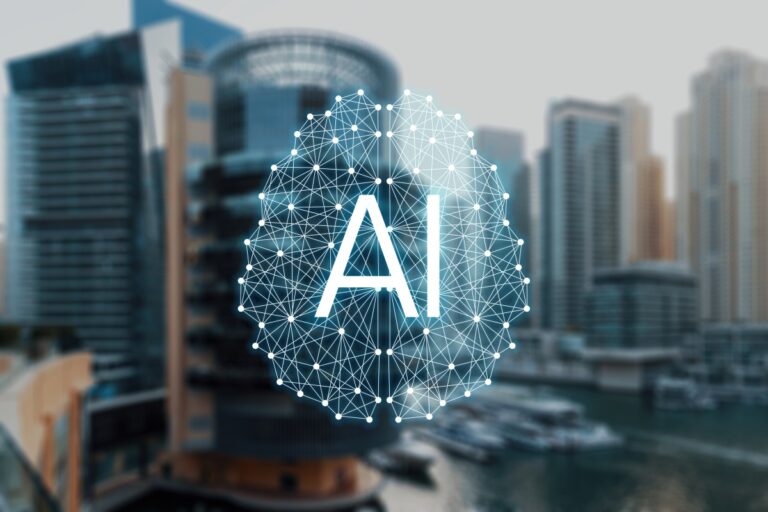
The Next Step for AI Research
Academic research must rely on a clear research agenda leveraging the latest technologies for innovative applications. Hence it is essential to analyze the next research challenge in AI – that is, integrating Machine Learning (ML), Knowledge Graphs (KG), and Multi-Agent Systems (MAS).
Artificial Intelligence and Analytics (AIA) are closely integrated to help automate processes and support decision making in all sectors of the economy. The past five years have witnessed significant investments in pilot projects geared toward transforming key public services, especially at operational levels (e.g., award-winning projects by the AI Labs at Immigration, Refugees, and Citizenship Canada and the Canada Revenue Agency). This has opened the opportunity for renewed partnerships between government business and technology experts, aimed at co-developing the next generation of analytics-driven e-government.
Machine Learning (ML)
Most of the implementation efforts in AI have focused on ML and its applications to extend the Data Science (DS) capabilities of large ministries and agencies. This was spurred by the rapid adoption of and experimentation with open-source development languages (e.g,. R and Python), and increasingly process-oriented big data platforms (e.g., Spark, MLflow, Kubeflow). While most results have been impressive and encouraging, there is a need to develop a broader and more forward-looking AIA strategy which will seek to maximize value for governments and carefully invest IT budgets and learning.
As ML may have been one of the “low-hanging fruits”, government DS teams are called upon to learn and integrate more complex AI technologies. Two that are closely linked to ML should be on the radar of all Chief Information Officers (CIOs) and Chief Data Officers (CDOs): KG and MAS.
Knowledge Graphs (KG)
Unstructured information, in both text and multimedia formats, are abundant in all public services. However, Governments have long invested in meta-data and indexing systems but have only scratched the surface of innovative opportunities to leverage this data.
KG technologies (e.g., Resource Description Framework, RDF; RDF Schema; Web Ontology Language, OWL2) are essential for the next step in AIA effectiveness. As one of the many variants of the NoSQL database paradigm, graphs provide more flexibility to represent complex relationships among entities and their data, as well as more versatile query mechanisms and algorithms. Hence, by enabling collaborative development of standard vocabularies and “ontologies”, KG technologies integrate several layers of information and contents management platforms, while giving new opportunities for knowledge extraction and decision support.
Multi-Agent Systems (MAS)
Robotic Process Automation (RPA) has been a focus of several governments, especially to better manage performance and risks in high volume processes. The public sector is likely to follow the same evolution as other sectors have followed, for example manufacturing. In the 1990s and 2000s, industrial production and supply chain integration triggered transitions between several generations of RPA: Business Process Reengineering (BPR), Lean Management, Business Process Management (BPM), Holonic Manufacturing, and Industry 4.0 as its most complete architecture.
MAS technologies are key to enabling more real-time RPA and seamless Human-Computer Interaction (HCI) in manufacturing, and public services will surely benefit from such advances. Intelligent Agents, an algorithmic and event-driven component programmed to handle low-level automated decisions, are the next step in RPA as they bring a degree of knowledge-driven autonomy in low-level repetitive decisions where criteria are clear and data is unambiguous (hence, the word “robotic”).
Integrated together, agents can be programmed to interact in a multi-agent community where they can query one-another, share resources for punctual tasks, take initiative as per pre-established rules, and especially relieve human intervention from the tedious and error-prone process of performance and risk monitoring.
AIA as an Integrated Architecture
The integration of ML, KG, and MAS technologies is inevitable. As it has been demonstrated in other sectors, DS models create an abundance of decision rules that can be later formalized as part of knowledge repositories. These can further be enhanced in a graph system to enable query and reasoning, helping to automate decision-making. Finally, the inference, rules, and reasoning systems can feed into a multi-agent environment, which in turn can feedback the inference and rules functionality through adversarial learning among agents.
While Industry 4.0 has yielded impressive results, the Government 4.0 movement should by far surpass the level of complexity, innovation, and impact of AIA investments, primarily for the sheer extent of public services, and their greater diversity of operational conditions. It is urgent that CIOs rethink their perception and understanding of the AI wave, and embark a more robustly architected vehicle ready to benefit from the next coming waves.
About The Author
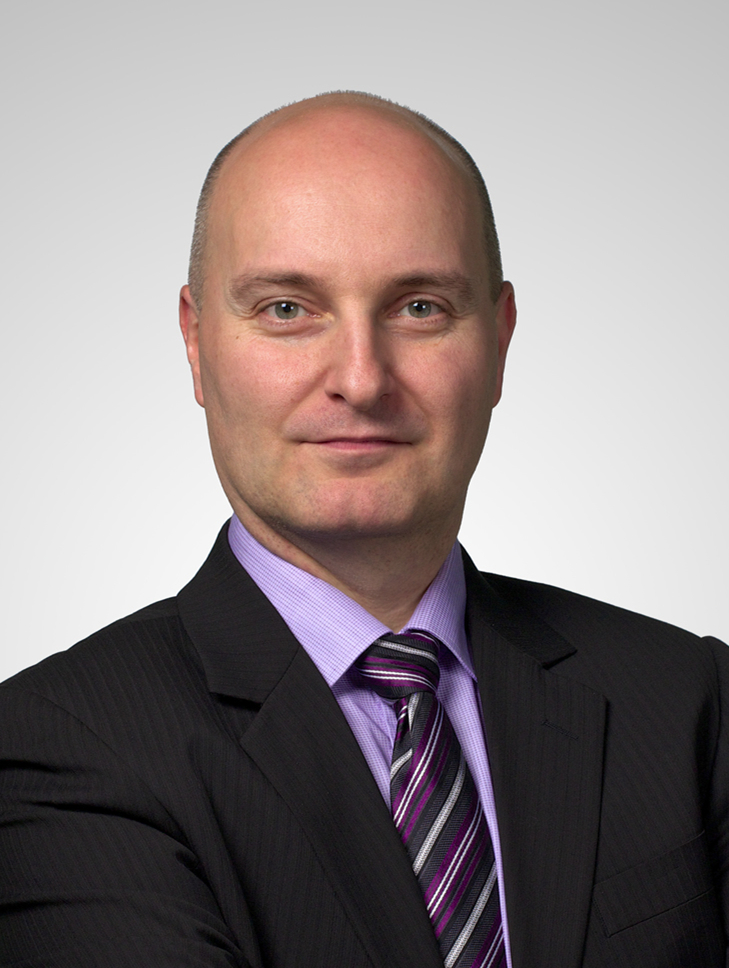
Stéphane Gagnon, Ph.D.
Stéphane is an Associate Professor in Business Technology Management (BTM) at the Université du Québec en Outaouais (UQO), based in Gatineau, National Capital Region. He is also a founding member of the Government Analytics Research Institute, a consortium between Carleton University, University of Ottawa, the UQO, Institute on Governance and SAS Canada.