ARTIFICIAL INTELLIGENCE
Artificial Intelligence Governance: An Operational Challenge
Despite many actors’ attempts to define principles for responsible use of AI, nothing substantial has been achieved, except loosely-stated fashion principles. However, the authors did not identify the factors that may have contributed to the absence of AI governance framework nor what is required to establish such a framework.
By Hubert Laferrière
![Use 'AI' to ensure [data security]](https://governmentanalytics.institute/wp-content/uploads/2020/02/trace-3157431_1920-768x512.jpg)
Absence of AI Governance
In their 2020 State of AI report, “ … a compilation of the most interesting things … about the state of AI and its implication for the future.” N. Benaich and I. Hogarth, two AI investors, mentioned the only prediction among the six they made in 2019 that did not materialize is AI governance. For them, the necessity for governance is sine qua non given the increasing power of AI systems and interest of public authorities. Despite many actors’ attempts to define principles for responsible use of AI, nothing substantial has been achieved, except loosely-stated fashion principles.[1] However, the authors did not identify the factors that may have contributed to the absence of AI governance framework nor what is required to establish such a framework. For example, should AI governance be underpinned by a body of law like the EU General Data Protection Regulation?
Responsible Use of AI
Yet, over the past years, many players have published proposals for the governance of AI, ranging from high-level principles to more down-to-earth directives. In Canada, the Toronto Declaration (Protecting the right to equality in machine learning) and the Montréal Declaration (For a responsible use of AI ) were published in 2018 with the aim of developing a responsible use of AI.[2] Both declarations are the result of collaborative work between partners from different backgrounds.
The Government of Canada led the development of AI guiding principles, adopted by leading digital nations in the same year to ensure a responsible use of AI while supporting service improvement goals.[3] Five guiding principles were established: understand and measure the impact of using AI; be transparent about how and when AI is used; provide meaningful explanations about AI decision making; share source code, training data, while protecting personal information; and government employees have the skills to make AI-based public services better.
The principles intersect with the major trends identified by the Berkman Klein Center at Harvard University in a comparative analysis of 36 principles documents aimed at providing normative guidance regarding AI-based systems. Each document had a similar basic intent: to present a vision for the governance of AI. The documents were authored by actors coming from different sectors of the public and civil societies, such as governments and intergovernmental organizations, private sector firms, professional associations, advocacy groups, and multi-stakeholder initiatives. Although the goals sought among them were different, the Centre has identified eight key or common thematic trends that rally the stakeholders. The trends comprise ethical and human rights-based principles that could guide the development and use of the AI technologies (privacy, accountability, safety and security, transparency and explainability, fairness and non-discrimination, human control of technology, professional responsibility, and promotion of human values).[4] For the Center, conversation around principled AI is converging towards a responsible development of AI. “Thus, these themes may represent the “normative core” of a principle-based approach to AI ethics and governance.” [5]
Explaining the absence of AI governance, as Benaich and Hogarth assert, is not a straightforward undertaking since a wide range of factors could be considered. For instance, one may argue that this absence is the result of a divergence of views between the public and private sectors. Some AI leading players in the private sector prefer to adopt their own guiding principles rather than having to comply with AI principles endorsed by public authorities or governments. Others may bring forward the proliferation, in the public and civil sectors arena, of committees, working groups, boards and commissions on AI and ethics. Despite good intentions, this proliferation has not yet generated an enabling AI governance framework. Discussions are too often limited to insiders and have yet to come to fruition.
However, stating that there is an absence of AI governance does not entirely reflect the reality of organizations using AI technology.
Managing AI
Those adopting the AI technology, in particular predictive analytics, machine learning, neural network, and deep learning, implemented management frameworks to support the development and the production of AI solutions. The management of AI activities is based on specific practices, processes, methods, and procedures related to the technology. Tools, such as the data science lifecycle and standardized methodologies such as CRISP-MD and ASUM-DM [6], are assisting AI practitioners and management to shape activities and processes, including performance monitoring and assessment. The tools support efforts to address unique AI issues such as training data, algorithms bias, and the drifting of AI models, to name a few. As a result, organizational and managerial efforts focus on setting the conditions for facilitating the technology to operate and produce value. The very AGQ first issue was on “Responsible AI” and provided a series of considerations to move forward. [7]
Two Spheres, Two Solitudes
In this context, the AI governance seems split in two spheres: (1) managing per se the dynamics (development and production) of the new technology, what is unique and specific to it and, (2) managing the impact of the technology, in particular issues and concerns raised by stakeholders, including clients affected by this technology. This impact is calling for ethical and human rights-based principles and legal frameworks.
These spheres have their own distinct interest and activities. I suggest that recognizing this situation could help to shed some light on the absence (at least the slow pace) of AI governance as observed in the 2020 State of AI report.
The problem resides in these spheres evolving in parallel, having their own management processes and procedures and, accordingly, being developed separately as two solitudes. This leads to a lack of integration or what has been identified as an absence of AI governance. In addition, each sphere cannot by itself offer an AI governance framework that will support efforts in maximizing the benefits and minimizing the harms of AI. Bridging or interlocking both spheres becomes an imperative.
Efforts to bring together the two spheres could be a path for developing a sound AI governance framework. This path must enable the achievement of a precise objective: ensure guiding principles and directives are transposed into a modus operandi for AI practitioners, too often stuck with second guessing the principles’ meaning and aim.
A Challenge
Meshing the two spheres is a challenge that must be tackled by the AI community, in particular the AI practitioners, and the key stakeholders of public and civil societies including governments.
Guiding principles may provide a sense of direction but often they are too general, subjected to a plethora of opinions, while abstract by essence. This renders the efforts and attempts to embed the principles into AI processes laborious and inefficient. Building the bridge between the AI day-to-day practices sphere and the requirements of the guiding principles sphere is not a given. This even if some principles are already enshrined in laws and policies, for example the privacy laws, current requirements may be restrictive, potentially stifling the technology, and probably not adapted to the new reality of AI.
Embedding Operational Practices into AI Business Process
AI practitioners need to embed the principles into operational practices and into components that will make up the DNA of their operations. AI practitioners carry out complex tasks and operations and need to outline operational practices which allow the integration of a guiding principle into the AI business process, in their AI day-to-day activities. The operational practice represents a roadmap for building the bridge that interlocks the two spheres. An operational practice contributes to create efficiencies, to ensure the AI solution is aligned with guiding principles and comply with the law. An operational practice brings consistency and reliability to produce an efficient and quality AI solution.
To illustrate that such an endeavour can be done, I am exhibiting a portion of the work undertaken by my ex-colleagues at Immigration, Refugees and Citizenship Canada and Prodago, a firm hired to assist them.[8] On the drawing presented below, the guiding principles form the foundation on which the three columns of the temple rest. For example, the privacy principle is selected. The team looked at The Privacy Act and related policies and directives to break them down into specific operational practices. The team adopted a Privacy by Design approach to carry out the efforts.
Outlined by the team at outset, these practices are implemented at the deployment phase of the AI business process. This means that an AI solution cannot be implemented for production if clear responses about compliance are not provided. The exercise must be done for each principle.
Establishing AI governance must be based on what characterizes the new technology and its own challenges. AI managers and practitioners cannot avoid the imperative of aligning their actions with key principles. In public service, conformity with laws and policies is a must. Lack of attention or care to this area will plague AI trustworthiness.
The establishment of operational practices that integrate AI procedures and methods with specific ethical (and legal) principles helps to build a sound governance framework. A bridge is built which is likely to pave the way for AI governance.
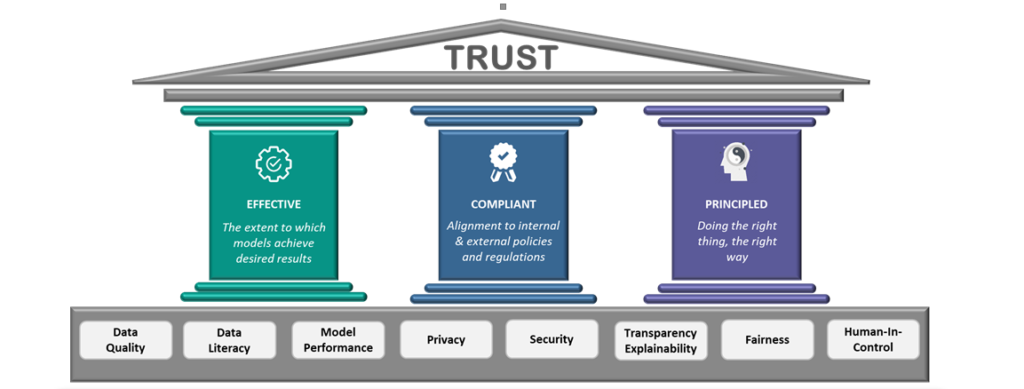
- N. Benaich, I. Hogarth (2020), State of AI Report 2020
- Amnesty International, Access Now (2018), The Toronto Declaration, Protecting the right to equality in machine learning and Montreal University (2020).
- Treasury Board of Canada Secretariat (2019), Ensuring responsible use of artificial intelligence to improve government services for Canadians.
- J.Fjeld, N. Nele, H. Hilligoss, A. Nagy, M. Madhulika, Principled Artificial Intelligence: Mapping Consensus in Ethical and Rights-Based Approaches to Principles for AI (January 15, 2020).
- Ibid., p. 5
- “Cross-industry standard process for data mining, known as CRISP-DM, is an open standard process model that describes common approaches used by data mining experts. It is the most widely-used analytics model. In 2015, IBM released a new methodology called Analytics Solutions Unified Method for Data Mining/Predictive Analytics (also known as ASUM-DM) which refines and extends CRISP-DM.” (extract from Wikipedia).
- Analytics In Government Quarterly For Government Decision Makers (2019), Government Analytics Research Institute (GARI).
- Thanks to Na Guan, Jae-Jin Ryu and Wassim El-Kass, my ex-colleagues at Immigration, Refugees and Citizenship for the use of the drawings and to Mario Cantin from Prodago for his substantial contribution. Gartner just recently recognized the firm as a “2020 Cool-Vendor” in AI governance.
About The Author
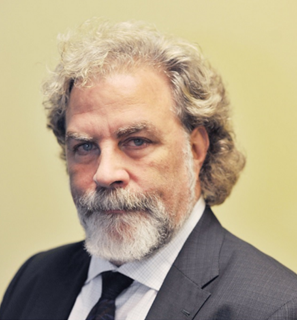
Hubert Laferrière
Hubert was the Director of the Advanced Analytics Solution Centre (A2SC) at Immigration, Refugees and Citizenship Canada. He had established the A2SC for the Department of IRCC and led a major transformative project where advanced analytics and machine learning were used to augment and automate decision-making for key business processes.