DATA SECURITY
Data Governance Prevention and Cure of “Fake” Data
Within the parameters of a governance framework organizations should have a Data Governance Committee. The authority of this committee should include oversight regarding the operationalization of data management and data integrity.
By Betty Ann M. Turpin, Ph.D.
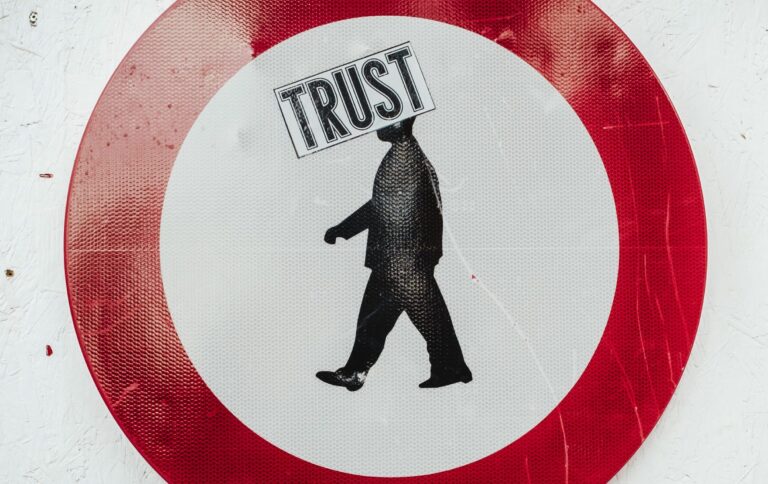
You have likely heard the expression “garbage in = garbage out”. Data are facts, figures, images, etc. at their lowest level of unit that are then aggregated to conduct analysis, visualization, and reporting – the latter from which interpretation follows – thus creating information. It is this information that is used for decision-making, program design, communication, learning, etc. Hence the importance of “good” data cannot be over-stated.
Within the parameters of a governance framework organizations should have a Data Governance Committee. The authority of this committee should include oversight regarding the operationalization of data management and data integrity. Obviously, a committee should not manage the operations, but Data Stewards, for example, within each business unit can. Fast forward to how to prevent and cure fake data, which hopefully provides useful insights for Data Stewards and organizations.
Typical reasons for fake data are:
- Deliberate misleading, often undertaken for personal /organizational gain of some sort;[1][2]
- Lack of knowledge of data techniques and skills, such as tidy data, analysis methods, and data understanding;[3][4]
- Poor data credibility.[5] Credibility refers to the quality of data being believable or trustworthy, the research methodology, and data sources. It includes the following data elements: integrity, quality, reality, context, and probity. These are affected by systems and humans;
- Poor due diligence, such as not fact checking or lack of concern about credibility. Sometimes this can be a function of “rushing” to get the information out, hence unintentional errors in the data.
So, what are some tips on how to prevent and cure fake data?
Suggestions for Prevention
Governance committees can guide the develop of policies and procedures to ensure fake data is minimized.
Data protection[6] and privacy[7] is a growing concern today. Protection is usually managed through network security protocols designed to prevent any unauthorized user, application, service or device from accessing network data such as secure file transfer protocol (SFTP), secure hypertext transfer protocol (HTTPS), firewalls, email/spam protection, secure socket layer (SSL), etc. Organizations do/can adopt security strategies, particularly important when data is shared between business units, and between government bodies such as the extensive collaboration within the Canadian Federal Government.[8] This helps keep unwanted hackers out and ensures that unauthorized users cannot change the data.
Data privacy is about ensuring that the personal information collected on individuals is secure and accurate. The latter is increasingly a concern, because as individuals attempt to ensure their privacy is maintained they may intentionally provide wrong data. This leads to erroneous information. In some situations, offering the individual an exchange of value (e.g., white paper, free access to trial software) for their information can prompt more accurate data provision.
Credible data practices go a long way toward producing and using “good” data. Data credibility can be supported by regularly screening the data to ensure data records are as accurate as possible. Consistency in implementing data standards, procedures and protocols is vital.
Machine learning can expedite the data checking process by automating the often-time-consuming task.
Suggestions for a Cure
Assume your data is untrustworthy until this assumption is proven false or you can trust the source or system from which the data is derived.
Be more discerning about the data and information you are using or relying. Look to trusted sources.
Be critical of the data you use or receive. Check the source, who else is reporting on this data or information, and be sure the data is credible. Use common sense, if it “looks” odd or seems unlikely, this is often a good clue as the potentiality of fake data – investigate and do not hesitate to ask the tough questions.
Theories provide testable hypotheses on which to assess the information produced by the data. Where they do not formally exist, only those with alternative explanations can challenge the data facts or information. Both forces enable us to look deeper into the data to look for inadequacies or inaccuracies. Artificial intelligence modelling using advanced data analytics can support this depth probing too, by specifying data rules and conditions.
Data, as a qualitative and quantitative unit by itself is not meaningful, until it is analyzed and interpreted, but even then it must usually be compared to something else, or historical data of the same units.
The more systematic and prior knowledge organizations have regarding the data in question, the better. This will enable data comparison to historical data or similar data.
Upskill by ensuring all your employees have a good understanding of data and the skills required to use data credibly. This means building a data culture within the organization. In today’s world, this is an imperative.
In closing, for a myriad of reasons, “fake” data is a growing concern for organizations, businesses, and citizens globally. This paper has not dwelled on the consequences or treatment of fake data or those who generate it. But I do leave you with a few questions: Should the consequences for creating and/or presenting FAKE data be regulated? What consequences can organizations impose in a timely, equitable, and legal manner?
- Kupferschmidt, Kai. (2018). Tide of Lies. Science. Downloaded from the Internet September 28
- Reyes-Velarde, A. (2020) Beware the coronavirus scams: Colloidal silver, herb remedies and fake test kits. March 22.
- Harris, Jeanne. (2012). Data Is Useless Without the Skills to Analyze It. Harvard Business Review. September 13.
- Wickham, Hadley. (2014). Tidy Data. The Journal of Statistical Software, vol. 59.
- Credibilty is a term is often mistakenly used interchangeably with integrity or evidence. The former is an element of credibility, the latter refers to information (means information bearing on whether a belief or proposition is true/false, valid/invalid, warranted/unsupported. Evidence alone is not sufficient to determine truth, it must be interpreted.
- AGJ Systems & Networks. (2020). Beyond Foundational Network Security.
- Pritchard, K. (2017) How do marketers solve the problem of fake data? Global Marketing Allicance.
- Public Safety Canada. (2020). Cyber Security in the Canadian Federal Government.
About The Author
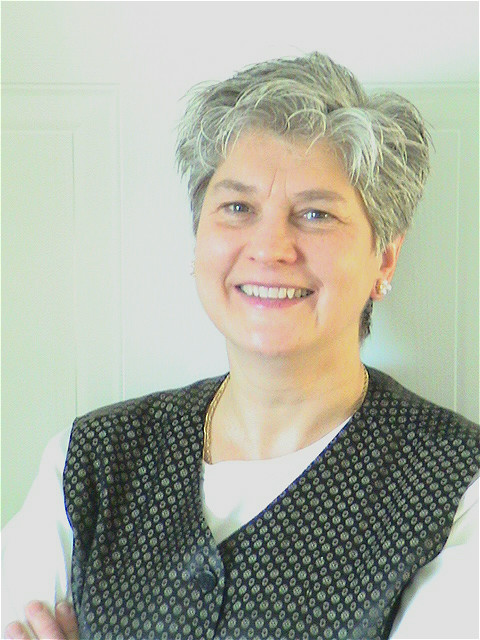
Betty Ann M. Turpin, Ph.D.
Betty Ann M. Turpin, Ph.D., C.E., President of Turpin Consultants Inc., is a freelance management consultant, practicing for over 25 years, has also worked in the federal government, in healthcare institutions, and as a university lecturer. Her career focus is performance measurement, data analytics, evaluation, and research. She is a certified evaluator and coach.