ARTIFICIAL INTELLIGENCE
Institutionalized AI: A Governance Conundrum?
Although it’s a very complex area, most government organizations already have the tools in place: business cases, performance measurement, and audit.
By Gregory Richards, Ph.D.
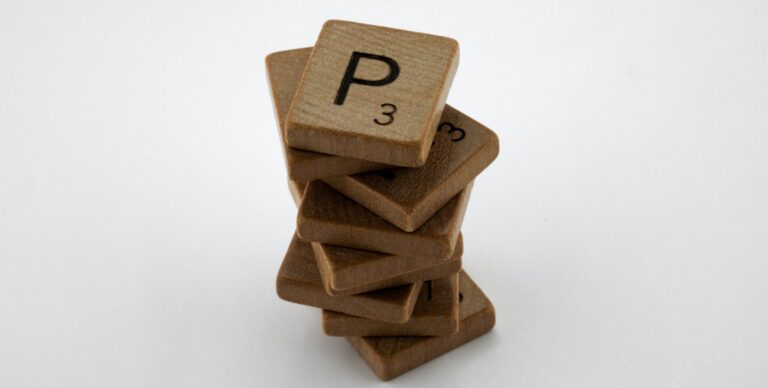
I am going to follow up on my colleague Hubert Laferrière’s discussion on AI governance. Although it’s a very complex area, I would argue that government organizations already have tools in place to enable sound governance of AI.
It’s no secret that Artificial intelligence (AI) has captured the imagination of government with visions of streamlined business processes and decision-making aided by algorithms. There are, of course the usual caveats about potential bias and privacy issues related to AI. But a broader question that has so far not been addressed is this: what governance structures should you think about when institutionalizing AI—that is, when integrating AI into the day-to-day business of your organizations? Should you seek informed consent from those whose data you are using? To what degree should they be aware of what you are doing with data you collect? How would you guard against bias when it comes to decisions that affect people’s work life? Although these are complex issues, the government already has tools in place to address them although a few tweaks are needed to tackle AI-related issues.
By way of context, research by McKinsey Global Institute suggests that the best approach for delivering value through AI is to integrate it into your day-to-day management processes. This notion is supported by the rationale advanced by Ajay Agrawal and his colleagues at the University of Toronto that AI is a sophisticated form of predictive analytics. Predictive analytics, like other forms of analytics, depends on data and data governance structures that have been well-established in the information systems field. But AI introduces some additional governance challenges that data governance structures did not anticipate.
Taking a broader view, we might ask ourselves, what is good governance? Many governance models exist, take for instance, The United Nations Economic and Social Commission for Asia and the Pacific that identifies the following eight characteristics:
- participatory;
- consensus oriented;
- accountable;
- transparent;
- responsive;
- effective and efficient;
- equitable and inclusive; and
- follows the rule of law.
Consider, for example, that you have integrated AI into your HR planning processes to identify which of your employees might retire or leave the organization in the next few years. To do so, you would use aggregate data to create the predictive model, but since the data are gathered from your employee base, do you need informed consent?
If you examine the eight characteristics above, the answer would be yes. Furthermore, as Hubert has mentioned elsewhere in this issue, the Berkman Klein Centre for Internet and Society at Harvard University summarized a variety of recommendations for principled AI in a report (published January 15, 2020) entitled Principled Artificial Intelligence: Mapping Consensus in Ethical Rights-based Approaches to Principles for AI. In this report (pg. 5), the authors conclude that the following three key themes are important for the use of AI:
“Privacy. Principles under this theme stand for the idea that AI systems should respect individuals’ privacy, both in the use of data for the development of technological systems and by providing impacted people with agency over their data and decisions made with it. Privacy principles are present in 97% of documents in the dataset.
Accountability. This theme includes principles concerning the importance of mechanisms to ensure that accountability for the impacts of AI systems is appropriately distributed, and that adequate remedies are provided. Accountability principles are present in 97% of documents in the dataset.
Safety and Security. These principles express requirements that AI systems be safe, performing as intended, and also secure, resistant to being compromised by unauthorized parties. Safety and Security principles are present in 81% of documents in the dataset.”
Every manager I’ve talked with about these issues agrees with the principles discussed above. The question is how to apply them, especially in a government context.
Fortunately, most government organizations already have the tools in place: business cases, performance measurement, and audit. These simply need to be modified to fit with an AI-driven organization.
The Treasury Board of Canada Secretariat (TBS) has published a Business Case Guide that outlines the basics: business need, options comparisons, and risk management. For an AI-driven organization, these sections would include and evaluate options that might not include the use of AI. If an AI approach is selected, then the section on risk would evaluate risks and potential costs related to data leakage, ensuring informed consent and managing security breaches. The overall cost-benefit analysis would consider total costs including those related to potential breaches and the cost of transparency.
TBS’s Directive on Results provides guidance for performance measurement. In the case of AI, the process is as important as the results achieved, and thus process-level performance indicators would need to be included in the organization’s results management framework.
Similarly, the TBS Policy on Internal Audit provides broad guidelines that would need to be expanded to include reviews of the operations of the AI algorithm including the “drift” associated with the algorithm over time and its potential for bias. The challenge here is the “black box” nature of most algorithms.
At the moment, AI adoption is in early phases, but as organizations move forward with integrating these tools into their day-to-day management processes, it’s important to rely on established policies and frameworks to ensure sound governance. For example, research is being done on “white-box” AI systems that permit users insight into the data being used and the way the algorithms treat the data. The consideration of these types of approaches would start with the business case and flow through performance measurement to audit.
Although the integration of AI is indeed complex, if we consider it to be an advanced form of predictive analytics, we can find ways to leverage current governance tools to better institutionalize AI into the day-to-day work of managing government organizations.
About The Author
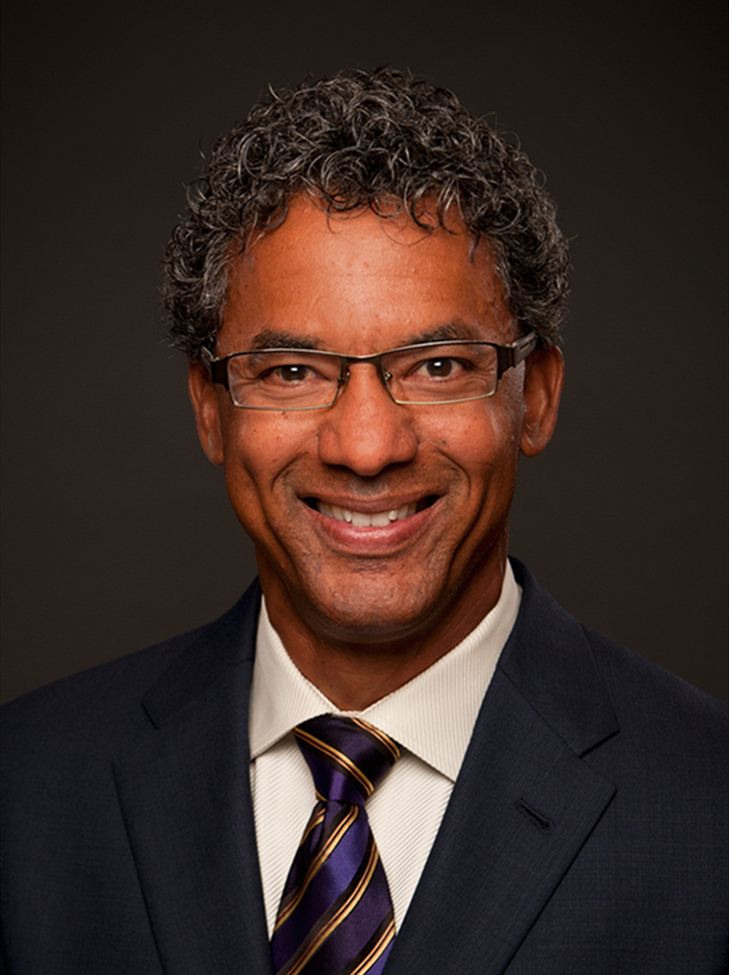
Gregory Richards, MBA, Ph.D., FCMC
Gregory is currently the Executive MBA Director and & Interim Vice-Dean, Undergraduate and Professional Graduate Programs at the Telfer School of Management at University of Ottawa. He was a visiting professor at the Western Management Development Centre in Denver, Colorado and a member of Peter Senge’s Society for Organizational Learning based at MIT. His research focuses on the use of analytics to generate usable organizational knowledge.