BIG DATA
Program Integrity, Entity Resolution and a 360-degree View of the Citizen
The lack of a citizen identity consistency and the integrity of such data has created challenges, and techniques like entity resolution can help.
By Amanda Holden & Dan Finerty
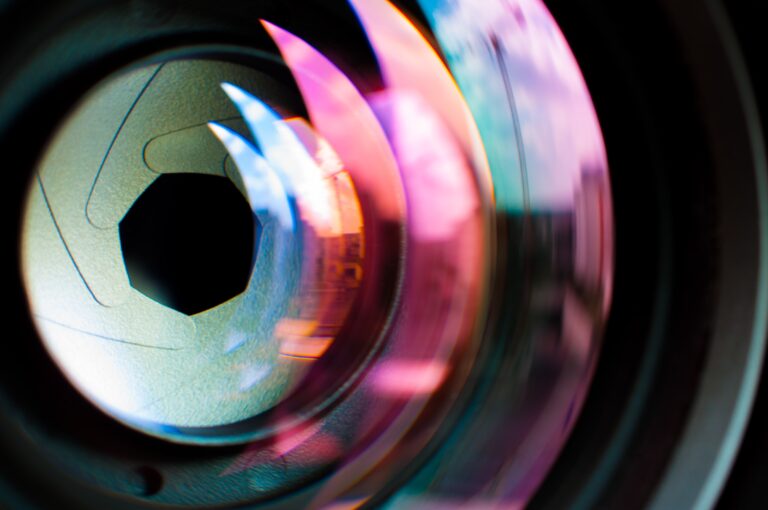
In late January, the first Canadian was diagnosed with a novel coronavirus. Scientists had been tracking the spread of the virus, but despite previous experience with outbreaks like SARS and swine flu, the scope and depth of the impact of COVID-19 was unprecedented.
Within weeks, business closures and quarantines would throw millions of Canadians into precarious financial positions. All levels of government scrambled to put together massive social programs on an unprecedented timeline.
Speed of delivery is always a prime consideration with any government programming. But responsible use of government resources is often a competing interest—how do we ensure services are delivered only to those who qualify?
This problem is exacerbated when multiple departments and levels of government are involved in the delivery. Canada’s response to the pandemic on a program delivery basis has been enviable, but not without hiccups. By June, 190,000 Canadians had to return payments made under the Canadian Emergency Response Benefit (CERB) program, often because they were unknowingly covered under another Covid-19 program—both Service Canada (through Employment Insurance) and the Canada Revenue Agency (CRA) are administering COVID-19-related programming. In addition, the Ministry of Employment, Workforce Development and Disability Inclusion estimated that one to two percent of claims filed were fraudulent.
These issues highlight fundamental challenges in program integrity—the lack of a citizen identity consistency and the integrity of such data has created challenges, and techniques like entity resolution can help.
Entity Resolution
The goal of entity resolution seems simple: Make sure you are dealing with the person you think you’re dealing with. It isn’t. The nightmare scenario for entity resolution is Robert James Smith.
First, there are countless Robert Smiths. Are we dealing with Robert Smith the professor, the politician, the war hero, the plumber?
Second, in various contexts, Mr. Smith could be identified as Robert, Rob or Bob. Suppose the Robert Smith in question prefers to go by his second name. He could now also be James, Jim or even Jack. These identities could emerge variously in passport applications, driver’s licenses, criminal records, employment records, professional registrations, business ownership records, property tax records… the list goes on. Some even use multiple identities intentionally, creating confusion so they can take advantage of the system.
Entity resolution is important because it is about mitigating the risks of improper or incomplete identification. And it’s more than just name checking.
The 360 View
Suppose for the sake of argument we’ve resolved the identity of the person of interest as Robert James Smith, the plumber. (We’ll go into detail about how later.) We know who we’re dealing with. But do we know what we’re dealing with?
The holistic, or 360-degree, view of the citizen comes from all the representations of identity, across departments and levels of government. Financial institutions have pioneered this approach to customer identity in the name of risk management. Is it safe to give this person a $50,000 loan? Consider his repayment record on credit cards. Does he have a mortgage, and thus security? Student loans? Savings accounts? Insurance?
Similarly, by pulling together Robert Smith the plumber’s interactions with vehicle licensing agencies, the CRA, municipal tax departments, EI, provincial and federal service agencies, etc., we can glean a more complete view of the citizen—and the likelihood of his eligibility for government assistance, or to attempt to defraud the program. Thus, the data needs to not only be managed, but requires a governance model.
Data Architecture
The integrity of this data is paramount. For example, in a pilot project that SAS Institute participated with in Ontario, some of the children were older than their mothers. That’s a rather serious data integrity issue.
At this stage of the process, we’re not concerned with what the data means, only that it can be trusted. It’s not about what the data says, it’s about whether it’s speaking the right language.
Data cleansing highlights anomalies (like children older than their mothers) and incomplete identities. It also prepares data to be integrated across systems. Fields that co-relate can be matched. One system’s Surname is another system’s Last Name, to cite a very simple example; phone numbers can be collected in myriad formats. Varying taxonomies can be reconciled for consumption across systems.
Citizen Protection
With data in a more manageable state, protecting the information—and the citizen—can be a more focused effort. It’s a three-legged stool:
Security. Tales of customer information being lost, leaked and stolen are nothing new. Robust data security measures must be in place to protect and secure data for appropriate access and use.
Privacy. Much data in the individual datasets, for example sensitive health information, shouldn’t be shared with other systems. That doesn’t mean data can’t be shared; insights and risks in the data can be shared in appropriate ways while still respecting privacy requirements.
Authentication. There is myriad data about Robert J. Smith in his online interaction (IP address, biometric behaviors, etc.) that can safely be used to confirm and protect the real Robert J. Smith. A consistent and deep 360-degree view of the citizen can protect the individual and government in many ways:
- With appropriate security, privacy and authentication controls, ministries can feel more comfortable sharing data across services and create a better view of what the individual needs and should have access to. For instance, an AI model might predict that the individual would benefit from a particular training program if they’ve recently applied for EI.
- Sadly, identity theft and falsified information are key criminal tools to defraud government programs. Accurate and a deep understanding of a citizen’s interactions can help AI to predict identity theft, protecting the citizen and the government from fraud.
- Entity resolution can be complemented with techniques like network analytics, which use even broader aspects of data to connect individuals across a network. This perspective allows ministries to see who’s connected to high-risk situations, creating a proactive view of interactions that can predict and prevent fraud and abuse.
Technology can be an impediment or an enabler. Rules baked into the data structure are needed to serve these three goals. With well-organized and quality data, policies, rules and practices can be automated to reduce risks.
Resolving Robert Smith
So how do we know we’re dealing with Robert James Smith (the plumber)? There is some golden entity resolving data, though some of it can lead us into the woods.
The gold standard is the social insurance number (SIN). If Robert Smith and Jack Smith have the same SIN, it’s almost indisputable that we’re dealing with the same entity. Also, other government departments reuse SIN numbers in their own systems; for example, the Ontario Health Insurance Plan (OHIP) bases its registrations on a variation of the citizen’s SIN. If these numbers don’t match, we may be dealing with two different people—or someone concocting an identity.
Addresses are also compelling evidence, but not as foolproof. Perhaps Robert Smith pays property taxes on a residence at 123 Any Street. To his customers and business partners, he’s Jack Smith, and applies for business relief under that name listing his shop address. This takes some untangling of the data. This is also true of phone numbers, perhaps more so—home, office, mobile phones credited to Robert, Bob and Jack could belong to the same person.
Beyond these basics, there are many other data points to help resolve Robert Smith: birth dates, other registration data, digital authentication data, and more.
How do analytics and data science streamline service delivery? Consider the case of CERB. As of September 28, the federal government had received 27,570,000 CERB applications from 8.9 million unique applicants. Entity resolution can pre-screen applicants for fast-tracking, while flagging anomalies for investigation—a triage, essentially. The 360 degree view of the citizen that cross-checks Jack Smith’s employment data, tax filings, business data, could flag him for further investigation.
Doing Even More
Deep analytics and artificial intelligence offer fertile ground for even further streamlining government programming. There is such a huge body of data to train artificial intelligence to discover and predict anomalies based on behaviour in a citizen interaction, and further focus investigative resources while speeding delivery. Better citizen outcomes with efficient and effective use of resources is the mandate of every government depart. Getting control of your data and exercising deep, program-related analytics based on input from program experts can make it happen.
About The Authors
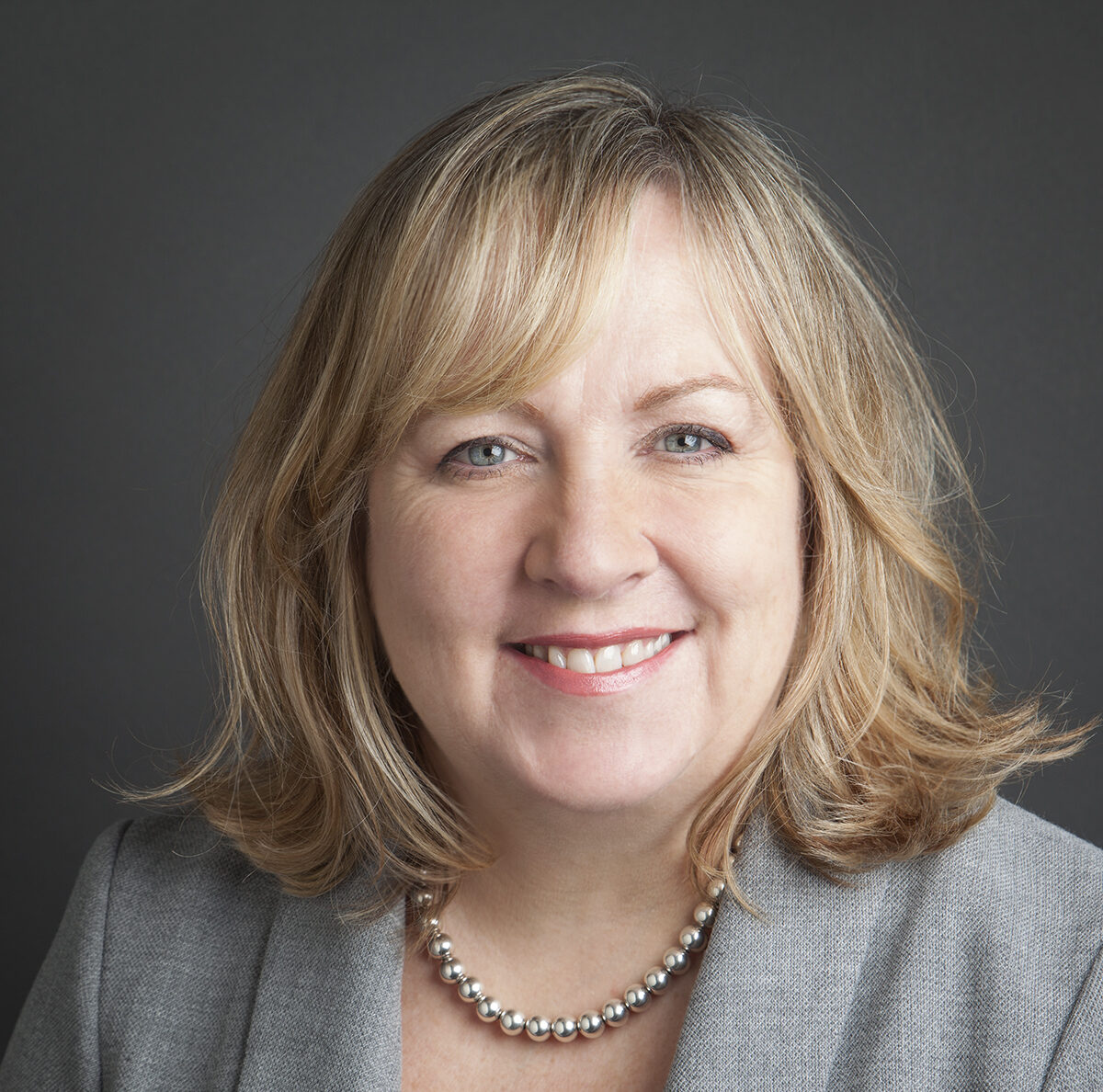
Amanda Holden
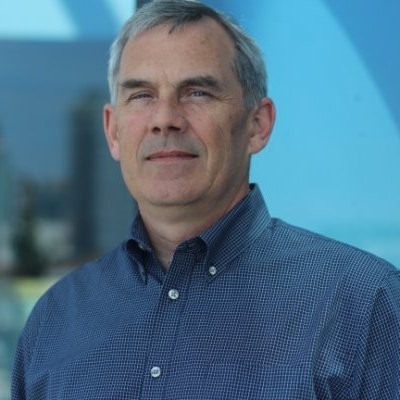