MACHINE LEARNING
Expanding Big Data Measures and Machine Learning to Manage Pandemics
With the synchronicity of multiple data sets and moderator variables, machine learning analysis capability can enhance predicative potential of pandemic occurrences and insight into what effective interventions can predict, prevent, and manage the viruses.
By Betty Ann M. Turpin, Ph.D.
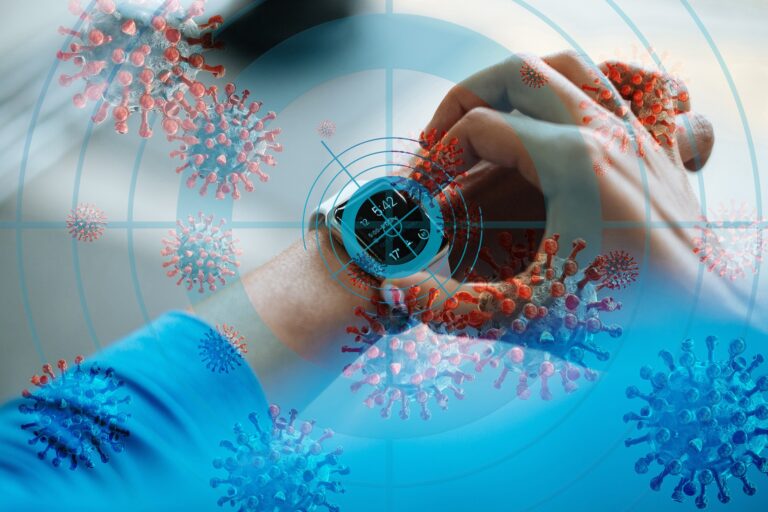
The world has pulled together remarkably well to combat the outbreak. To understand this pandemic, nations have turned to public health and infectious disease experts. As we struggle to gain control, a primary and understandable focus has been on the direct causal effects related to contracting and transmitting the virus. Based on information releases and expert opinions, there is a high degree of consensus that nations will have to manage this outbreak for at least 18-24 months in the hopes of it subsiding and stabilizing, as researchers try to find a cure.
Globally, countries are reporting data such as the number of cases, recovery and death volumes, and even indirect data such as flight travel patterns. However, each country is using its own methodology for identification, data gathering, analysis, and reporting. Time has not permitted the development of a global data cloud for data sharing. Big data sources would yield valuable insights but will require global harmonization and standardization of the data methodology. A good example of cooperation can be found in the Canadian Public Health Surveillance initiative that collects information related to 29 specific diseases and threats to public health in sentinel systems to track and forecast health events and determinants. [1] Currently Canada, through the Public Health Agency of Canada, is coordinating with the provinces and territories, and using the current flu tracking system, to track and update on the virus. The measures are comprised of 7 components [2]: geographic spread, laboratory confirmed detections, syndromic, outbreak, severe outcomes, strain characterization and antiviral resistance testing, and vaccine monitoring. These components are lagging indicators/measures that are also used to generate forecasting data.
Some countries have begun to consider the secondary impacts of COVID-19, such as deaths due to transplant surgeries not being possible, lower than average blood supply so that lives cannot be saved, starvation rates due to increased lack of access to food, to name just a few. However, capturing this information is not easy and is certainly resource intensive, and again varies across nations. These are consequences of the disease occurrence but suggest variables that could be used in prediction and management of pandemics.
So, if we are to look at the “health” of a nation, during the peak and recovery periods, and even the prevention of COVID-19 re-occurrence, we need to know why specific interventions work (i.e., what are the underlying mechanisms) and for whom and under what circumstances produce the greatest benefits. To do this, we must look beyond direct causal effects and lagging indicators and consider an indirect-relationship model, one that includes mediating and moderating variables that influence uncontrolled and controlled variables. Using an indirect-relationship model can improve the understanding of the pandemic and thus its management.
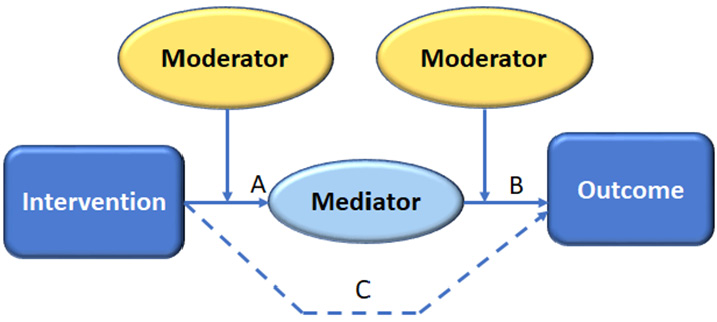
As shown in Figure 1, a mediator as an intervening variable that is affected by the intervention (line A), which in turn affects the outcome that is desired (line B). Conceptually, mediator models assume that the intervention causes changes in the mediator variable, and the mediator variable then causes changes in the outcome. But other factors (other variables) become apparent. Moderators are qualitative (e.g., sex, race, class) or quantitative (e.g., the level of reward) variables that affect the direction and/or strength of the relation and relate to personal situations, psychological profiles, and work differently for different types of people.
A moderator variable (vertical arrows) affects the direction (+/-) or strength of the relationship between the intervention and the mediator, or the mediator and the outcome. Line C is an unknown variable that may/not affect the outcome.
For example, consider the current-day interventions adopted in most countries. Physical distancing is the intervention, as the assumption has been this intervention will lower transmission rates. The mediator is social behavior. Thus, the desired outcome is the reduced spread of the virus. So, to identify moderators, we ask the questions: 1) what might affect the relationship between the intervention and the mediator, 2) what might affect the relationship between the mediator and outcome. In terms of the first, age is a factor and may be a stronger relationship for older adults (50+ years) and less strong for young adults, access (to stores, parks, etc.) and wearing facial masks are the second moderators. But at this point in time, because we do not collect or integrate this information into the data analysis, we can only assume they contribute to a positive outcome.
Importantly there can also be/unknown/unanticipated variables that impact (line C) the outcome. Such as defiance of the distancing recommendation, refusal to use a tracking/tracing app on one’s phone, etc. To identify variable C, it would be helpful to consider research work related to unintended consequences.[4] Additionally, this indirect casual model shows just one outcome, but there be many, and so for each outcome, this model should be applied.
We know this intervention and variables have already been applied in most countries and is working.
We also know that many surveillance systems track moderator A-type variables, and most of these are lagging indicators. The challenge to expanding surveillance is to identify other moderator B variables and then build these into the AI system. The focus should be on interventions that yield the best and strongest effects, that is, can moderate the outcome. A second challenge is knowing what these variables are/may be. We do not always know, so applying the unintended consequences approach can help.
With the synchronicity of multiple data sets and moderator variables, machine learning analysis capability can enhance predicative potential of pandemic occurrences and insight into what effective interventions can predict, prevent, and manage the viruses.
- https://www.canada.ca/en/public-health/services/surveillance.html.
- https://www.canada.ca/en/public-health/services/diseases/flu-influenza/influenza-surveillance/about-fluwatch.html
- Donaldson, S.I. Mediator and Moderator Analysis in Program Development. In Sussman (Ed.), Handbook of Program Development for Health Behaviour Research and Practice (pp. 470-500).
- Morrell, Jonathan A. (2005). Why Are There Unintended Consequences of Program Action, and What Are the Implications for Doing Evaluation? American Journal of Evaluation.
About The Author
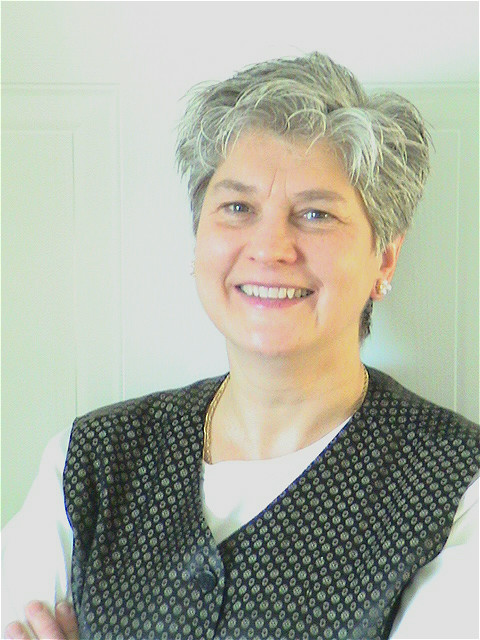
Betty Ann M. Turpin, Ph.D.
Betty Ann M. Turpin, Ph.D., C.E., President of Turpin Consultants Inc., is a freelance management consultant, practicing for over 25 years, has also worked in the federal government, in healthcare institutions, and as a university lecturer. Her career focus is performance measurement, data analytics, evaluation, and research. She is a certified evaluator and coach.