FEATURES
Climbing the Mountain: Automated Decisions for Government
Governments must make a fundamental shift for AI and analytics to fuel a post-pandemic rebound, from technology adoption mindset to a decision impact mindset
By Tara Holland
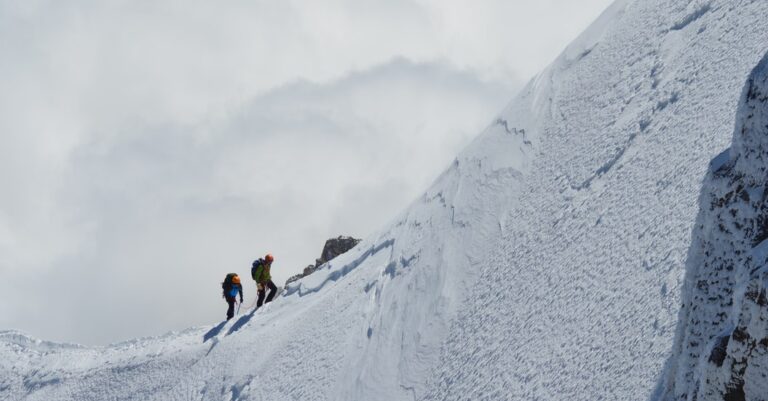
Data analytics and artificial intelligence have long proven their value in terms impact on business outcomes. There are many concrete examples of the impact of analytics and AI in the Canadian public sector.
- The Centre for Addiction and Mental Health (CAMH) uses predictive modeling to co-ordinate 34,000 patients across 90 independent mental health services.
- The Ministry of Alberta Justice and Solicitor General use analytics to comb through silos of data, cross-referencing related people, places, and events, across police, courts and corrections jurisdictions.
- Canada Post is incorporating analytics to drive cost savings, improve marketing campaigns, and improve customer relations.
But most are predictive and pattern modeling efforts which can mean insufficient actions is taken on the data, and the extent of follow through is questionable. There are greater potential benefits when AI and automation have a direct impact on frontline decisions.
“By 2024, 60% of government AI and data analytics investments aim to directly impact real-time operational decisions and outcomes,” according to research firm Gartner Inc[1].
Automated decision-making is real-time, at scale, and natively digital. More importantly, it is business-led and is inherent in policy and program design.
Pivoting to this new paradigm can be daunting, more so for the public sector than private sector — the public sector has many more stakeholders, more complex measures of success, slow uptake on digital solutions, and a more risk averse relationship with change.
When faced with what feels a daunting task, perhaps we can draw lessons from another discipline: Mountain-climbing.
Mountain-climbing is a very arduous and risky discipline—there have been an estimated 280 deaths on Everest alone. When climbing the automated decision-making mountain, we can emulate mountain-climbers proper preparation to minimize the risk and increase our likelihood of success.
Mountain climbers prepare for an expedition in three ways: physically, technically and mentally.
Physically. Obviously, you want to in your best physical condition. It’s not just about rock-hard abs and powerful biceps. Multi-stage climbs require acclimatization to altitude, a strong cardio-pulmonary system, strength, balance, and coordination. Preparatory climbs can begin weeding out climbers whose physical constitution isn’t up to the task.
For real-time automated decision-making, your data must be physically ready. Ensuring data credibility is the preparatory foundation that your data must pass. “Dirty data”— outdated lists, missing fields, inconsistent formatting—leads to errors, delays, and, in a public sector context, health and safety issues and liability exposure.
There are many dimensions to data quality measurement needed to verify data credibility:
- Accuracy measures the degree to which data values are correct.
- Completeness means all data elements have tangible values.
- Consistency focuses on uniformity of data.
- Timeliness to ensure the most fresh and current data.
- Uniqueness validates that each record or element is represented once within a data set, helping avoid duplicates.
Data quality management identifies data that isn’t up to the task and good data management practices build the physical readiness needed for automated decision-making.
Technically. Sir Edmond Hillary and Sherpa Tenzing Norgay didn’t make it to the top of Everest alone. The expedition—two teams of climbers—were supported by 362 porters, 20 Sherpa guides, along with food, shelter, clothing and climbing equipment.
That 10,000 pounds of baggage was necessary because the equipment of the time was primitive by today’s standards. Today’s climbers have lighter options: parkas with lighter synthetic fill, strong nylon rope, lighter yet more effective shelter, redesigned technical equipment made of lighter metal.
A technical infrastructure for automated decision-making consists of many components. One that is responsive and quickly implemented.
- High-volume, high-speed computing power. Just as climbing equipment has evolved so has the ways in which governments can access computing capacity. Cloud providers provide the ability to more easily access and scale the resources needed for AI and automation.
- Low-code and no-code tools. Traditional analytics and AI development that required deep understanding of and the ability to develop using a coding language are too slow to respond to the needs of intelligent decisioning applications. For public sector organizations to take advantage of the decision-making knowledge and skills in their workforce, they must provide the modern tools to enable business users to develop the solutions.
Cloud computing and modern development tools are two of the important “lighter” technologies needed to successfully achieve automated decision-making.
Even if the equipment is lighter, Everest climbers still need sherpa guides. Government must look to the experience in other industries and the vendor community to provide the subject matter experts that can help guide the way and avoid the dangers. Too often, it’s tempting to read the manual and do it yourself. Everest climbers don’t carry their own equipment.
Mentally. Climbers spend about two-and-a-half months on Everest. That’s a long time to stay focused. Detailed planning, sometimes beginning years in advance, can help keep that focus on the mountain. Closely related is visualization, attempting to “see” or “feel” themselves performing techniques and strategies.
This focus allows them to avoid “summit fever,” an undisciplined rush to the peak that poses a danger to themselves and others.
The trek up your own Everest also requires thorough planning. Develop a road map to visualize the endeavour, and picture the process need to make the necessary organizational and cultural commitments to the project.
Integral to the organizational readiness for an automated decision-making strategy are governance and ethics. There are the explicit legal and regulatory responsibilities, but there are also questions like:
- How can data be shared—or not shared?
- What are the security and privacy implications?
- Do we have to collect more data for an accurate decision?
- How do we ensure that this data is unused in a fair, ethical and equitable matter?
Most importantly for governments, AI-driven, automated decision-making needs clear policies on where and how the program authorities oversee and ultimately own responsibility for the decisions.
The federal government’s Directive on Automated Decision-Making outlines the amount of human intervention required for a decision according to the nature of its impacts and duration of its impacts. You can turn to that for guidance.
The path to automated decision-making can be a long, arduous trek. But, like summiting a mountain, the reward is well worth the effort—faster, more effective, fairer public services and improved citizen outcomes.
Best of luck on your trek. We’ll see you at the summit.
For more information about the journey to automated decision-making, download the Canadian government e-book Transformational Decision
1.Gartner, Top Technology Trends in Government for 2021, by Analysts: Rick Howard, Bill Finnerty, Ben Kaner, Arthur Mickoleit, Michael Brown, Irma Fabular, Neville Cannon, Alia Mendonsa, Dean Lacheca, Apeksha Kaushik, Katell Thielemann, March 2021
2. Government of Canada, Directive on Automated Decision-Making, https://www.tbs-sct.gc.ca/pol/doc-eng.aspx?id=32592
About The Author
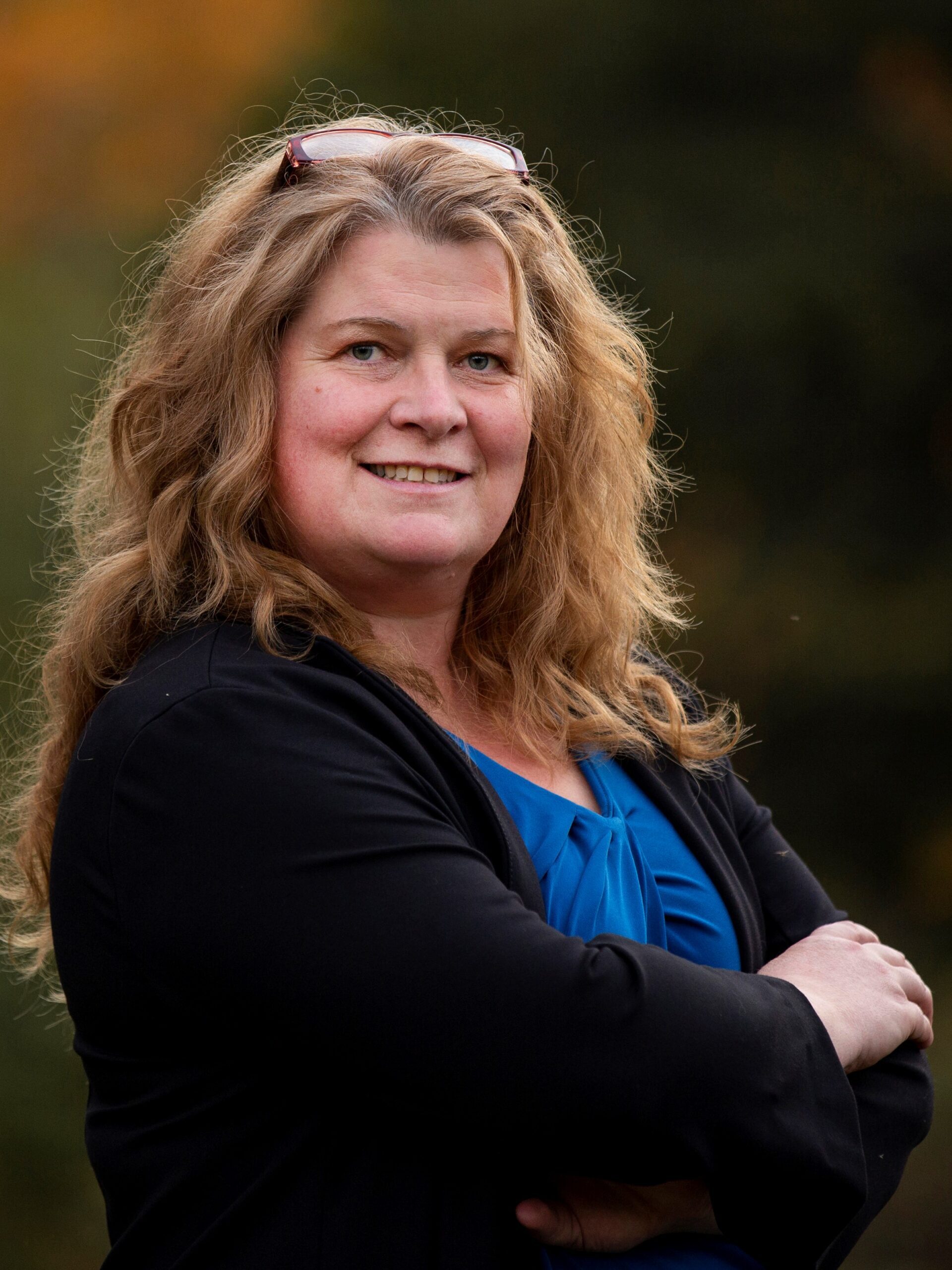
Tara Holland
As an industry specialist in the Global Government Practice, Tara brings best practice and proven solutions to government customers and partners around the world. Her role is to connect and collaborate with the leaders from the industry and across business units with in SAS. Based in Canada, Tara has been with SAS for over 20 years focused on supporting public sector customers. Her passion is in bridging the gap between business needs and technology solutions and enabling public sector organizations to deliver on the value of data. Prior to joining SAS, Tara led the Data Mining team at Canada Revenue Agency.