COLUNMS
Getting Data Science Started in Your Organization
By Kevin Kells
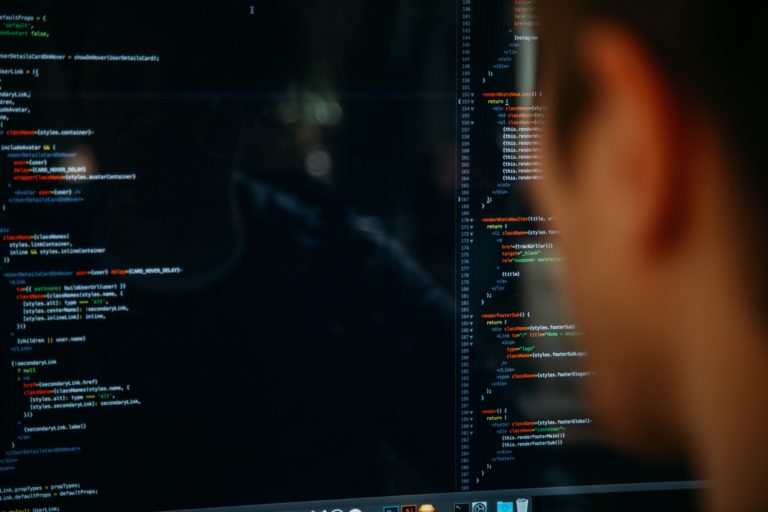
“I know the status of data science being used in my department. And I know where we’re headed. We have a realistic plan, taking gradual steps towards fully utilizing the data we produce. We are using data in ways to inform our entire organization from management down to guidance at street level for our caseworkers. Our employees are informed about it, feel engaged, and are excited about where we’re going. They’re happy to take part, especially those who have shown interest and curiosity and who have opted in to the online learning modules that we’ve made available to them. And those who are less technically inclined know they will benefit, too, without having to learn programming or statistics. Our stakeholders in the bigger picture are also satisfied with the multi-layered approach we’re taking to respect and protect the privacy and the confidentiality of the data we manage.”
Does this sound too good to be true? If so, it may be a relief to know that you are not alone. There are many organizations who also think the aspirations expressed in this statement are beyond the reality they are experiencing. They are hindered not just by available resources — both budgetary and human — but by a lack of clarity in big-picture understanding of what data science can be doing to help their organization and what they can be doing to grow their organization’s “data science maturity”. If your organization’s most advanced data science is — or until recently was — limited to Microsoft Excel, you are in good company.
“Data science maturity is a matter of a culture of learning, employee participation and technology. In that order of importance.”
The adoption of data science in an organization evades the linear principles of other changes, for example a change in technology tools rolled out by the IT department, a change in how business is done as driven by management, or a change in culture, as exemplified and promoted by leadership. The reason is that the adoption of data science involves a change in all three of these aspects at the same time. The introduction of new technology tools is just one of three aspects in data science adoption. The integration of data science into business processes and thinking is another aspect that involves those doing the work identifying how data can be used, and then adopting the use of that data in workflows and operations. Finally, there is a requirement in data science for a measure of openness to trial and error, a sense of exploration, and a touch of creativity.
These three aspects of data science adoption are depicted in the following graphic below. Here, the three aspects are laid out clearly. Let’s start by looking at the least important aspect, technology. This aspect involves the planning and installation of IT systems that can be used to store data, and software that can be used to analyze data and visually and interactively present results as information and knowledge. This technology aspect also includes the creation (by training and education) or importation (by hiring or outsourcing to consulting firms) of the knowledge in statistics, programming, and data science techniques required to properly transform data into meaningful and useful information and knowledge.
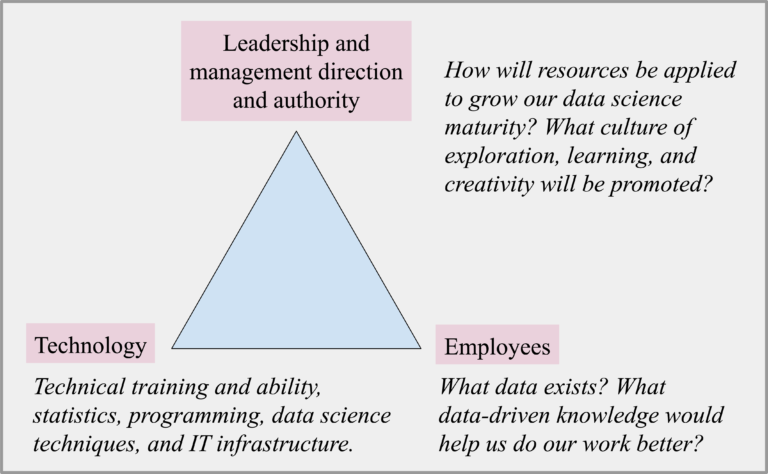
The next aspect involves the employees. It is not through technology, nor through leadership and management, that we answer these questions: 1) What data do we have, and how can we leverage it? 2) Given the available data, what kind of knowledge and information can be extracted that would relate to the daily needs of the work experience, be valuable to improving the quality and efficiency of work results and the quality of work life? The role of employee engagement, participation and input is key to answering these questions, and we’ll give some suggestions shortly about how to go about cultivating this input, no matter in what stage of data science maturity your organization finds itself.
Finally, the role of a culture of learning and exploration is a key concept to grasp, especially for the leadership and management, who may mistakenly consider the growth of data science maturity in the organization to be a matter of simply purchasing IT systems or outsourcing number crunching to a consulting firm. Why is learning the most important? Because it is through learning, exploration, and creativity that your employees — your organization — discovers what data is available that it hadn’t previously considered, how to extract knowledge and information from that data, and how that knowledge and information can be integrated into the daily experience, the operations, and the workflows throughout the organization. The factor of fun in enticing employees to engage in identification of data and how to use it should not be underrated. In fact, the concept of “gamification” in data science is gaining more and more recognition. It has little to do with “games” and much more to do with unlocking creativity, exploration, and discovery, and engaging end-users—your employees—in a way that for them is enjoyable and intellectually stimulating, and yet effective. If the participation of the employee is key to discovering an organization’s data and its most effective use, it is a culture of learning and exploration promoted by leadership, which will empower employees to participate.
What can an organization do?
The curious chasm can be bridged between management’s desire to grow an organization’s data science maturity and the role of the individual employee’s exploration and personal experience in growing that maturity — complicated by the third factor of technology — software, hardware, and knowledge of statistics, programming, and data science techniques.
Here are three action items to consider:
1. Promote an ecosystem for data science exploration among employees. Even without knowing which employees will step forward with ideas, let your organization know that leadership is deciding that there is likely latent data science curiosity, if not talent, and that managers, in an atmosphere of curiosity and discovery, should keep their eyes out to cultivate interest.
2. Invite employees at any level to take introductory online courses in data science, such as edX online courses (www.edx.org) organized by University of California Berkeley, M.I.T., Harvard, and other highly regarded universities. Your organization can sponsor a number of seats and reimburse employees for the cost, which, e.g., for UC Berkeley’s “Data 8X: Foundations of Data Science” on edX costs around US$ 270 per student for a 2-4 month course. Keep track of which of your employees sign up and complete the course, as they will be the first ones to follow up with to invite their ideas for how to apply what they’ve learned in the daily work of their teams.
3. Sponsor periodic data science summits within your organization, where employees or employee teams can showcase to the entire organization efforts and results from data science projects they’ve worked on within their departments. Winning recognition and even prizes for the best data science projects provides the rare benefit of moving all three aspects of the needle at the same time. First, it encourages employees to explore what data is available to them and what information or knowledge would be most meaningful for them to extract to incorporate in their work experience. Second, it highlights, to those wishing to get started, who in the organization has technical knowledge and technical resources and how to contact them to share and export that technology and those technology ideas to other parts of the organization. Finally, leadership sponsorship of data science summits within the organization sends an unmistakable message that exploration is encouraged and even rewarded.
An organization’s data science maturity relies on the balance of a three-part effort to progress. Invite your employees to explore and to propose which data they think is useful for them and how they’d like to see it analyzed and visualized. Support the employees with education and with the technology they request to work on the projects they propose that incorporate data science into their workflows. Reinforce the culture of discovery and exploration; data science talent among existing employees can be nurtured and their efforts to explore projects can be enabled and shared within the organization. This will not just inspire and empower other employees to learn and explore, it will create an atmosphere and ecosystem for data science discovery that will be attractive for hiring data-science-ready new talent.
Reference: www.mckinsey.com/business -functions/mckinsey-analytics/ our-insights/how-companies-are -using-big-data-and-analytics