Anonymity Is Key to Protecting Personal Information.
Government has an appetite for AI, but can’t expose PII. Why a sound governance strategy is crucial to data use.
By Arnold Toporowski
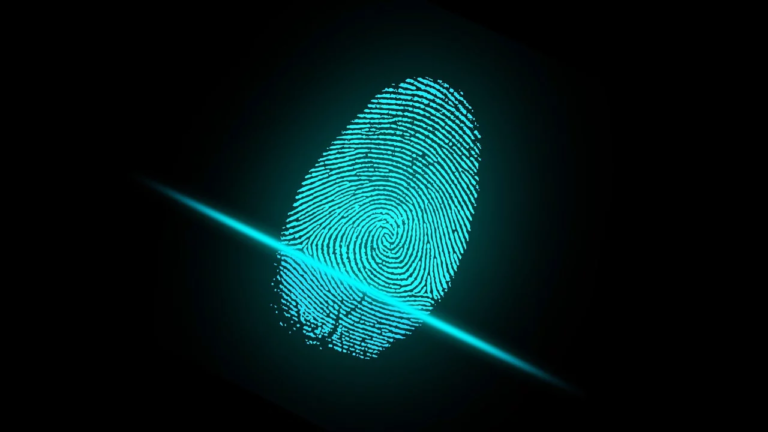
Governments are eager to explore the benefits of artificial intelligence. AI has the potential to streamline services and provide better risk management for government departments. But there’s also the potential to expose personally identifiable information (PII)—a violation of federal privacy legislation.
For example, the delivery of benefits like employment insurance and disability payments is a labor-intensive process. Applications and claims must be screened for eligibility (and possibly misuse). AI processing can speed the assessment of claims and improve the accuracy of service delivery.
Better risk assessment also promises improvements in efficiency and effectiveness. AI has a proven track record in risk assessment for financial institutions, one that could be applied to processes in the Canada Revenue Agency, for example. Zero-adjustment audits—audits that find no discrepancies—are frequent, and a drain on resources. Analytics that can identify higher-risk returns and prioritize them would allow auditors to spend their time more effectively.
Appetite for Data
If government has an appetite for AI, artificial intelligence has an appetite for data. Fortunately, that’s a hunger government can feed. With a body of 50-plus years of digitized records and a statistical agency that’s the envy of other nations, government is well-equipped to deliver on the data front. While the historical data may be incomplete by today’s standards, e.g., with new fields and data formats like video and audio, it’s a boon for machine learning (ML). ML is a key piece of the AI puzzle, allowing systems to learn patterns without human intervention.
There are many third parties who are interested in having access to government data and who would be able to provide government with valuable insights and results. Some government departments are very interested in making some data public for data science projects.
With the rise of post-secondary data science programs at Canadian universities, there’s an opportunity for government to share databases for post-graduate projects that can have an impact on service delivery.
But there’s a catch: The most useful data contains personally identifiable information that can’t be disclosed to third parties. The federal government’s Personal Information Protection and Electronic Documents Act (PIPEDA), along with similar legislation at the provincial level, governs how organizations can collect, use and disclose personal information. The use of PII is tightly controlled. One solution is to use aggregate data—data amalgamated from a large number of personal records with the PII omitted; while that may be useful for predicting trends in service usage, there are circumstances under which that’s not useful.
Anonymization
If government wants external data scientists to create models that can predict behavior of individuals, aggregate data isn’t useful.
Enter anonymization—redaction, encryption or obfuscation of PII that maintains the integrity of the individual record. It’s more complex than it sounds. The Canadian Institute of Health Information has said that that encryption of a patient’s health card number, name, address and telephone information is inadequate. An individual’s records can very likely be identified with just birth date and postal code alone.
This is called low-frequency data. Statistics Canada has a process in place to blank out such data. There’s a minimum threshold for the number of results returned by a query. Below that number, results are excluded. While Statistics Canada largely deals with aggregate data, it becomes more granular the further you drill down into a particular cell.
So how do you maintain protection of PII and still get useful results?
One approach is to broaden the scope of particular fields. For predictive power, a person’s age may be important, but the actual date of birth is not necessary. The address region may be significant, but the specific postal code might not be. Postal code K2J 1S8 is a stretch of houses on Greenbank Road in Nepean; the K2J forwarding station has 23,593 delivery locations. Using only the first three characters of the postal code makes personal identification much less likely. Anonymization is a sliding scale. This fuzziness of data helps protect PII.
Best Practices for Securing PII
Like anything related to information security, a PII governance strategy relies on three elements: people, processes, and technology.
People. A data governance committee, chaired by a chief data officer or chief information officer, establishes the principles of data use. How can the data be used? What data can be disseminated, and for what purposes? Who can use what data? This strategy must abide by the guiding principles of PIPEDA. Data stewards are responsible for ensuring access is according to the established protocols.
Process. The process for data use must secure critical information, but it must be usable as well. Automated processes should be guided by the Canadian federal government’s Directive on Automated Decision-Making, a first-of-its-kind framework for determining when human intervention is required in automated processes. At the same time, it can’t be overly bureaucratic—the objective is to streamline freeing up users for more value-added activity.
Technology. The right technology for governance is also critical. The data governance strategy must be embedded in the technology, automatically applying governance rules to access to data. For example, technologies like SAS Data Preparation and SAS Federation Server can govern data access and put in place secure views that prevent access to raw fields as necessary. Increasingly there are reports of stolen personal data from corporate systems showing that the exposure of personally identifiable information is a real risk. However, that mustn’t stop government from using data to provide better, faster and more effective services to its citizens. With a sound, well-designed governance framework embedded in processes and technology, and the promise of AI and machine-learning technologies to free up staff for more value-added activities than scrutinizing claims and applications, these opportunities can be exploited with controlled risk.
About The Author
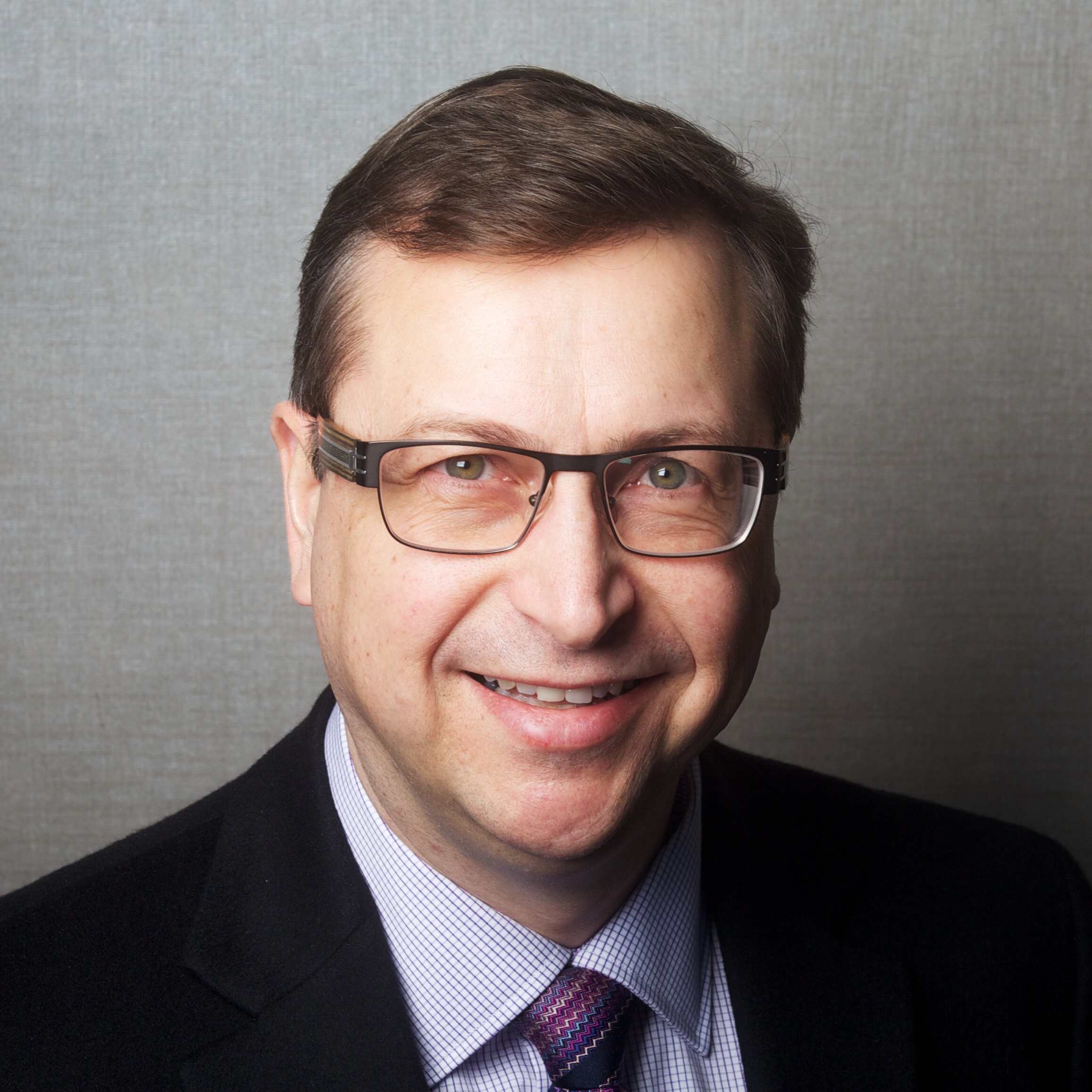
Arnold Toporowski,
Government Customer Advisory at SAS Canada, brings over 30 years of experience in Information Technology specializing in DataOps to the Government Customer Advisory Team.