FEATURES
Data validation in public sector human resource systems
By Sunil Meharia, MS, MBA and Betty Ann M. Turpin, Ph.d., C E.
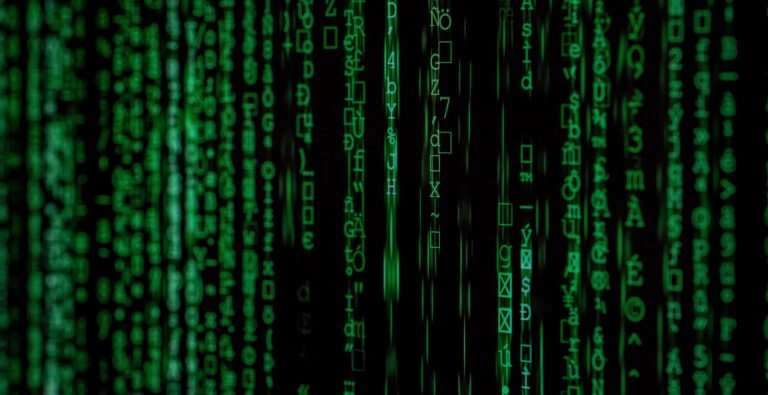
With the rapid onset of data analytics and artificial intelligence within the Canadian Public Sectors (Federal, Provincial, Territorial), organizations are scrambling to ramp-up their data processes, digital systems, and human resource (HR) complement and competencies. The latter has also given rise to a very competitive market for data analyst/scientist talent. It has also led to a transformation of HR management (HRM) in order to meet the growing demands of competitive hiring and provide fast, accurate data turnaround to support informed decision-making related to any aspect of the employee lifecycle.
HRM spans the continuum of the employee lifecycle (Figure 1) from hiring to departure, and is governed by several legislative and regulatory frameworks at all levels of government. With the ever-increasing need to better understand, predict and respond to workforce changes and requirements, it is paramount to understand how people move through their careers and identify effective methods of learning and the intricate relationships between people-related factors (e.g., employment equity, tenure, gender, sick leave, and multifactorial combinations of factors). This has reinforced the need for HRM to move from being transactional to a strategic decision-making partner, where HRM uses data to make evidence-based decisions and takes a proactive role in advising organizational business sectors on HR matters. One key challenge is the multiple sources of data (internal and external) that HRM needs to access. Most of these data sources are held by external GoC sources (e.g., PSPC), but some are based on the department/agency’s Peoplesoft data base (MYGCHR) (Figure 2). Several of these sources provide dashboards and tables related to the employee lifecycle.
Evidence-based decision-making necessarily relies on credible [1] data. Data analytics means carefully and logically thinking about the decision(s) that is required; systematically gathering, cleaning, and validating the data; interpreting the data, and reporting on the interpreted information to support informed decisions. The analytics process entails a typical data pipeline and should be the same process, regardless of the program or service area, and entails a typical data pipeline model (Figure 3).
HRM, as the custodian of the organization’s HR data, is responsible for its accuracy and usage and is in a unique position to contribute to the organization’s vision. To optimize data use, data management and analytical capacities must be enhanced. It will also require enhancing and developing new technologies, tools, policies and procedures, and re-engineering business processes.
In most Public Sector (PS) organizations, while HR units perform analytics, the processes involve a lot of manual workloads. Data entry within a PS organization is done by HR staff, employees, and managers, and as such data errors and/or untidy data occurs. Untidy data requires cleaning and correcting which can be manually laborious and very time-consuming. This is particularly relevant when separate data sets are integrated. For example, currently, within the Canadian Federal PS, there are nine fragmented HR systems that departments/agencies extract data from, and sometimes the data in various systems are not provided in real-time, or methods of analysis differ from what the department/agency requires.
Untidy data can result in issues during the reconciliation of data between different HR systems or within the HRIS. The Audit of Human Resource Data Integrity was conducted as part of Correctional Service Canada’s (CSC) Internal Audit Branch (IAB) in 2011. Since some data fields were not accurate, the Salary Management System could not be reconciled with the HRMS. One of the instances where this situation occurred was when an employee was funded by one cost center but was actually working in another functional area. This impacted all downstream HR metrics and often resulted in inaccurate KPIs.
Data validation seeks to uncover data errors or untidy data. It is an automated process that enables the user to check the data for accuracy, completeness, and formatting. This process ensures that the database maintains “clean” data. Data validation should occur at every step along the employee lifecycle.
Due to restrictions or lack of inter-portability of data within various HR Systems, data collected in one system often may not be transmittable to other systems. It is not advisable to manually input the same data across different systems as it increases the potential for data entry errors and redundancy. However, this is often done because the means (e.g., programming, APIs, AI) to automatically integrate the data is lacking.
To overcome this challenge, HRM must have the ability to identify different data quality issues with a validation process that is automated, systematic, and periodic. Every HRM business unit will have its own contextualized rules for how data should be stored and maintained. To be more efficient and effective with data usage, setting basic data validation rules is necessary. The most critical rules used in data validation are rules that ensure data integrity. Once established, data values and structures can be compared against these defined rules to verify if data fields are within the required quality parameters.
To achieve efficient validation tests, with actionable insights and easy to build logic, it is necessary to start at the most granular level of HR Data. Data formatting has been one of the biggest challenges when multiple HR Systems are involved. The main focus of automation of the HR Data validation process is to establish a logic between different HR data attributes (such as Hire date, Seniority Date, Job function, etc.) to clearly understand whether it is meeting the business expectation or not. For example, the termination date of an employee cannot be equal to/or prior to the hiring date. This level of automation can be performed using a scripting language to specify conditions for the validation process. An XML file can be created with source and target database names, table names, and data columns (data attributes) to compare. The validation script will then take this XML as an input and process the results.
Based on an HR Technology stack, workflows can be created specifically for data validation or this data validation can be added as a step within other HR data integration workflows (Figure 4). Once developed, these workflows can be reused or could be scheduled. The user gets notified through a validation report or a dashboard if data is invalid. This helps in tracking data integrity issues and helps in data correction, especially when data sets need to be merged/combined.
In summary, automation of data validation ensures that there are no inconsistencies or errors (correct data), no missing fields where a value is expected (complete data) and aligned with corporate data definition (data compliance). Data validation logics should be continuously upgraded to support new data fields, any enhancement in data definition, and new data integrations.
Reference:
- Credible data evidence is derived from sound, accurate, and fair assessments of programs and must address: probity, context, reality, quality, integrity – thus it yields the qvuality of being believable or trustworthy.
- In untidy data, there will exist inconsistencies in the data file such as in variable names, observations are stored in columns when they should be in rows, dates (dd/mm/yy) are not in the same order, etc.
- Audit of HR Data Integrity: https://www.csc-scc.gc.ca/005/007/005007-2509-eng.shtml#ftn12
About The Authors
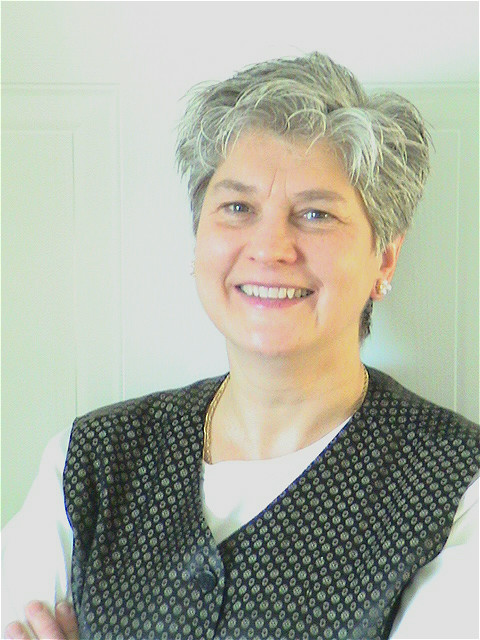
Betty Ann M. Turpin, Ph.D., C.E.,
Betty Ann M. Turpin, Ph.D., C.E., President of Turpin Consultants Inc., is a freelance management consultant, practicing for over 25 years, has also worked in the federal government, in healthcare institutions, and as a university lecturer. Her career focus is performance measurement, data analytics, evaluation, and research. She is a certified evaluator and coach.
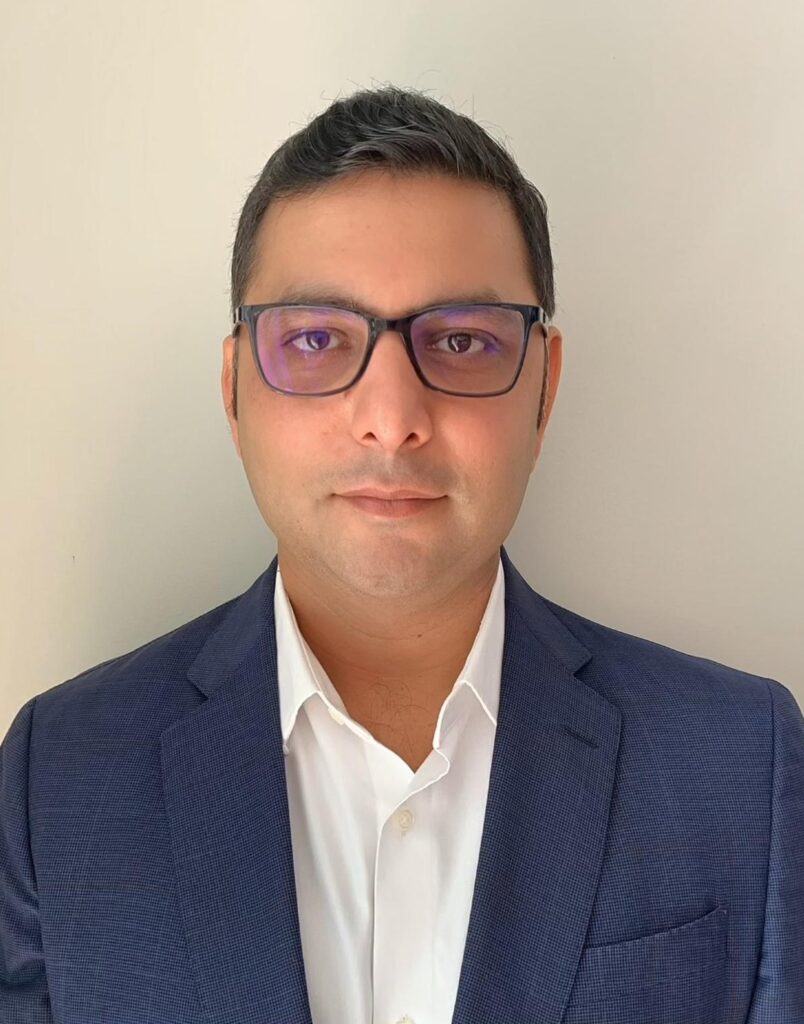
Sunil Meharia
Sunil Meharia is a HR Analytics Manager with a deep interest in how analytics can transform Human Resources. Over 12 years+ of experience across the Public sector, large manufacturing, and research-based conglomerates, his primary focus has been on data analytics in human resources, business strategy, and HR transformation with multiple years of experience in implementing Oracle HCM solutions for Fortune 500 companies. .