FEATURES
Is Human Oversight of Automated Decision System Unsolvable?
By Hubert Laferrière
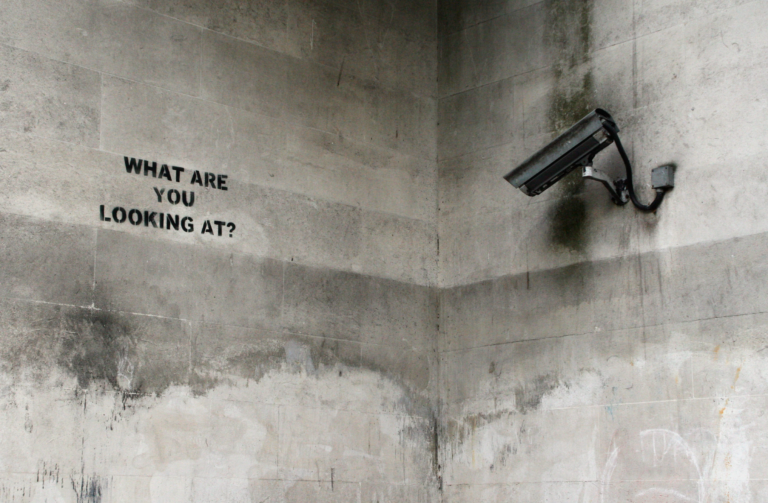
“Human-in-the-loop”, “human control of technology”, “human insight” are terms associated with automated decision system (ADS). They involve measures to avoid adverse or harmful effects on people. They have become more important in public sectors such as health, social welfare, taxation, police, and communication, because “harm reduction” pursuit has increased over the years and the means to deal with adverse effects are limited.
ADS is a decision-making process that uses machine and algorithm to automate processing without any human involvement. It may replace human judgement by generating a score (e.g., someone who reached the acceptable score is automatically granted of the benefits of a government program). Or it may assist an agent to perform administrative decisions by bringing in evidence based on factual data and inferred data. Some believe that the continued and increasing use of ADS by states affects governmental approaches to manage public services and functions and that perhaps the replacement of human judgment by an algorithm makes people more vulnerable. The UN High Commissioner Agency is concerned that the continued and increasing use of ADS by states pose negative, even catastrophic risks to human rights, policing and justice. Recent reports in Brazil state numerous errors generated by an algorithm trained on racial biases that has led to people being imprisoned when they should not be.
Addressing Harms: Human-in-Control, Human Insight
In Principled Artificial Intelligence: Mapping Consensus in Ethical and Rights-based Approaches to Principles for AI, the authors found “human control of technology” as one of the eight themes that constitute the “normative core” of a sound AI governance. They distinguished three key principles that embodied the theme: (1) the human review of automated decision principle; (2) the ability to opt out of automated decision; and (3) the opportunity for people to choose how and whether to delegate decisions to AI.
Government authorities have formulated, for some years, policies to regulate the use of ADS in the public sector. They defined the key requirements of the “human-in-the-loop” or “human insight” necessary to ensure that algorithms must do what it must do without adverse effects.
The Canadian government implemented, in early 2020, a Directive on Automated Decision-Making. All departments and agencies must apply the Directive and undertake an Algorithmic Impact Assessment. The risk level results determine additional and appropriate requirements such as quality assurance procedures and control measures. Thus, “human-in the loop,” which is listed as a mandatory item to be assessed, is being adjusted at the required level to mitigate risks: with minimal impact risk, decisions may be made automatically without direct human involvement. With higher impact risk, a decision cannot be made without having specific human intervention points throughout the decision-making process; the final decision must be made by a human.
The Automated Decision Systems Accountability Act of 2021, a legislative proposal currently in the process of being adopted by the California Legislature, would impose continuous tests for biases during the development and usage of the ADS. Earlier this year, the European Commission made a proposal for a regulation laying down harmonised rules on artificial intelligence, complementing the General Data Protection Regulation (GDPR). The proposal prescribes prohibiting unacceptable AI practices and banning AI systems that could potentially harm people.
There are already several calls for firmer measures: Michelle Bachelet, the UN human rights chief, called for a moratorium on the sale and use of AI systems, including a ban on AI applications that cannot be operated in compliance with international human rights law. Many cities in the US have shared the same line of thought: they had already banned the use of facial recognition systems used by police and security enforcement agencies because of many mishaps.
The policies are not only calls for rights and ethical principles of compliance and respect. It is an appeal for implementing specific measures to frame the algorithm itself and its operating conditions, this because an algorithmic chain value may hold several defects (up to nine social biases were identified). The policies include specific measures to prevent the negative effects of algorithms (mitigate or ideally end them). Absence or lack of consideration about measures safeguarding a true human insight or control over the algorithmic model is detrimental to the sustainability of ADS.
Flaws in Human Oversight Policies
Conducting a review of forty human oversight policies, Ben Green has found some flaws with the current and proposed control measures on algorithm. He has observed that policies were not based on solid evidence, and they were not sufficient to ensure proper human oversight. As a result, people are unable to perform the sought-after oversight function. Moreover, it engendered a more damaging consequence: the current human oversight policies legitimize government uses of faulty and controversial algorithms.
For instance, policies aiming “solely” at restricting ADS end up narrowing the scope of cases that could be problematic, leaving the faulty ones in operation. Several policies encourage bypassing some restrictions, like Article 22 of the GDPR (this situation is often referred as rubber stamping). Other policies encourage the work in tandem, algorithm and human judgment, allowing human judgment to overrule automated decision results; this may result in the decision-maker being bound to information generated by the ADS (automation bias/fettered decision). In the case of policies proposing a meaningful human oversight where human is the unique entity who can consider the context (e.g., a “subject” dealing with an ambiguous and conflicting situation), B. Green found the policies did not supply criteria nor standards to clearly define human oversight.
Reinforcing the Human Insight Framework
The findings and conclusions are worrisome as they cast serious doubts on the ability of policies to permit proper supervision and control over algorithms and their operating conditions. If ADS resumes its growth within public sector, the role of human insight becomes a more pressing issue. There is a need to develop more targeted and exact measures to reinforce a human control framework. Green proposes a twofold approach to delineate such measures.
First, policy developers, data scientists, executives, and program management must simply examine whether there is a need for ADS for their targeted business process. To do so, one needs to decide if the process relies only on human judgment. If it is the case, ADS is not appropriate. If there is no or little need to exercise human judgment (often labelled as discretionary judgement) and a thorough due diligence on the algorithm was undertaken, then, ADS use could go forward in tandem with human judgment or can even go forward solely. This is somewhat similar to the Canadian Directive about proportionate risk mitigation measures for the “human-in the loop” requirement.
Green will then insist on filling the information gap about the impact and interaction of the algorithm and human judgement. For instance, experiments on the collaboration between human and algorithm including ongoing quality assurance measures that could shed light on what is the core element of automated decision. This will generate empirical knowledge from the “inside.” In return, both information and knowledge could help policy developers to better align oversight policies with tangible and embedded measures that could be easily integrated to the algorithms.
Green recognizes his proposals are based on a technological solutionism approach. He is convinced that evidence-based frameworks “…develop approaches to using algorithms that promote rather than undermine central values of public governance.”
We barely scratched the human insight or in-the-loop question. One thing is sure: the need to scrutinize further the effects of algorithms on decision making, including interaction between human judgement and algorithm. This would allow better defined and more precise policies to avoid negative impact on people. Another “thing” is also sure: clearly confirm central values and develop practical criteria for public governance.
References:
- Turkle, S. Technology and Human Vulnerability; Harvard Business Review (2003), 81(9). And: Anderson, J. and Rainie, L. Artificial Intelligence and The Future of Humans (December 2018); Pew Research Center.
- The Right to privacy in the digital age, Report of the United Nations High Commissioner for Human Rights; (13 September 2021 – Advance Edited Version); A/HRC/48/31.
- Racisme. Au Brésil, les systèmes de reconnaissance des suspects posent problème; Courrier international (2021-10-10); Courrier international SA, Paris.
- AB-13 Public contracts: automated decision systems; (2021-2022); version 07/15/21; California Legislative Information.
- Proposal for a Regulation of The European Parliament and Of the Council, Laying Down Harmonised Rules on Artificial Intelligence (Artificial Intelligence Act) And Amending Certain Union Legislative Acts (April 2021), European Commission, COM (2021) 206 final.
- UN News, Sept.15 2021
- Silva, S and Kenney, M. Algorithms, Platforms, and Ethnic Bias; Communications of the Association for Computing Machinery (ACM), vol.6, number 11, pp.37-39, November 2019.
- Green, Ben, The Flaws of Policies Requiring Human Oversight of Government Algorithms (September 10, 2021). SSRN: https://ssrn.com/abstract=39212
About The Author
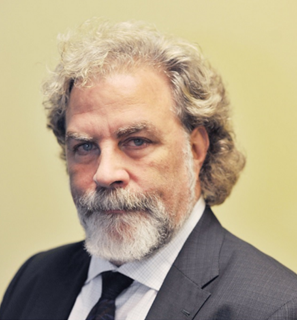
Hubert Laferrière
Hubert was the Director of the Advanced Analytics Solution Centre (A2SC) at Immigration, Refugees and Citizenship Canada. He had established the A2SC for the Department of IRCC and led a major transformative project where advanced analytics and machine learning were used to augment and automate decision-making for key business processes.