Artificial Intelligence and other advanced forms of analytics are new tools for many organizations. This inherent novelty means that researchers and practitioners have focused extensively on the technology to try to better understand how to use these tools. But, to fully integrate analytics into organizations, we need to consider that we are dealing with a socio-technical system: technology impacts the way we work even as the way we work influences how we use the technology.
Socio-technical concepts emerged out of research conducted in the late 1940s by the Tavistock Institute. The first observations were noted in coal mining. One interesting fact is that as mechanization of mining at the coal face progressed, individual workers found themselves more isolated. But as more advanced forms of mechanization were introduced building on the initial efforts, teams found that they were able to work collectively on complete tasks thus reducing isolation and improving working conditions. The underlying premise is that the implementation of any technological solution should consider the specifics of the human system it is supposed to enable.
This paper gathers thoughts from AGQ authors related to the introduction of advanced analytics in government organizations. What is the long run expectation of the use of analytics? Will organizations become more productive? Will we see the kind of “mechanization of management” noted in the initial Tavistock studies? Will the wide availability of analytic tools and processes democratize information in such a way that work teams can become simultaneously more collaborative and autonomous?
But I also think it will call for a rethinking of the process of management. Most organizations have already been moving in the direction of less “command and control”. In the government context, we cannot escape policy directives, but we could at some point, integrate regulatory structures within the analytic framework such that all decisions become “context aware” permitting more autonomy for work teams. Our research on regulatory intelligence, for example, suggests that in a few years we will be able to link program goals to specific regulatory instruments so that managers are continually aware of the policy context as they make decisions enabled by analytic tools.
Indeed, it is not “just” about the technology. But like in many other adoption cycles, it takes time before practitioners awaken to the importance of integrating social-technical systems. In the case of AI and Analytics (AIA), we should be thankful that this phase of “blind tech. euphoria” has been relatively shorter and less damaging than some others, for example, the e-commerce bubble from mid-1990’s, or the race for full-scale Enterprise Resource Planning (ERP) implementations of the early 2000’s. Also, the rigor that most governments have displayed in emphasizing ethical and explainable AIA, and dutifully implementing policies that follow world-class best practices, are all reassuring trends. The changes in AIA strategy in 2020-2021, linked obviously to the ominous spirit of the pandemic, have nevertheless laid a more solid ground for project success and scalability in coming years.
But some weaknesses remain, and as I wrote in the last issue of AGQ, our AIA strategies must evolve beyond simply focusing on Machine Learning (ML). There is a wide range of AI technologies, including Knowledge Representation, Knowledge Graphs, Decision Rules, Semantic Reasoning, Multi-Agent Systems, Robotic Process Automation, etc. All of these integrate with ML and Analytics, whether descriptive, predictive, or prescriptive. Again, it is not just about these technologies, but public sector AIA shops are bound to develop solid competencies in many of them, depending on the specific functionality required by their policies and jurisdictions.
In the long run, more government organizations will see the opportunities of using artificial intelligence in their operations. It just makes sense. Productivity is about doing more with less, and one of the resources that is becoming scarcer is human-response time to an ever-increased demand for our attention. In this deluge of data that needs to be analyzed, artificial intelligence algorithms will do most of the grunt work and leave the high-level analysis and decision-making to humans. That will allow them more time to work on the complex cases without being overwhelmed by too many easy-to-deal, simple decisions.
I don’t think that incorporating AI solutions will make our jobs more mechanical, on the contrary, by reducing the demand on our limited attention span, we can become more efficient and effective in our decision-making activities. To really benefit from these AI solutions, the data that governments have must be open, that way the AI algorithms will become more robust and accurate. Management will change, because everything else is changing, but the change is one that will make our organizations more agile and ready for the challenges of the future.
What will be the future for the public sector? I wish I had a good ML algorithm to predict it.
There is no doubt that using AI, in particular ML, to automate tasks and decisions will contribute to making public administration and service delivery more effective and efficient. Digital technologies are already currently playing a prominent role in shaping up and regulating the behaviors, performances, and standards of our world. The technology has already changed leadership and strategies, employee relations and trust, organizational change, and management science.
In this socio-technical landscape, the transformation brought about by AI, in fact by information technology in the public sector (Digital Era Governance, Digital Transformation, or e-Government), may attenuate or make disappear the respective limits between the public policy development and service delivery domains. If so, public policy is and will be gradually integrated into a service delivery logic, figured out by computational logic and by a techno-solutionism approach, often driven by practitioners who believe that their own technoscientific expertise is particularly relevant to the identified social problem. Indeed, the terms public policy engineering, computational public policy, political engineering, and computational politics, based on the application of engineering, computer science, mathematics, or natural science to solving problems in public policy. Is that a desirable outcome? The concern is like those aimed at the popular New Public Management approaches at the end of the last century. Some will say that, on the contrary, IT and AI allow for a better focus and precision on public policy formulation away from parochial interests and would contribute to service of public-spirited goals. I do not have an answer, and if I have one, it is incomplete. The questions are imperative.
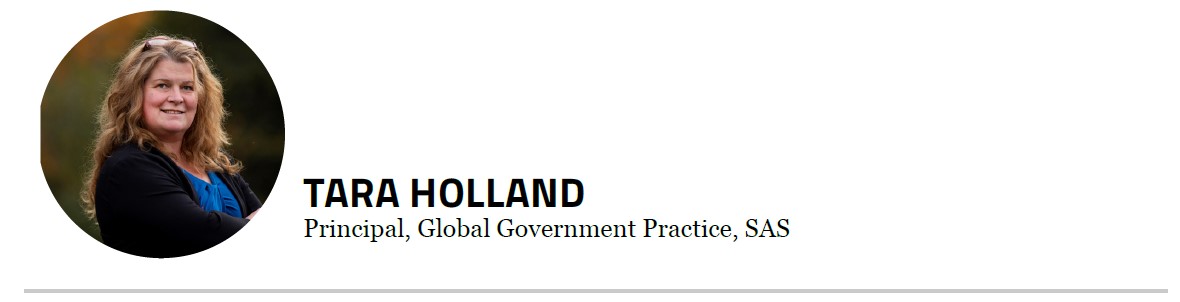
In the context of government mandates, it is important to recognize that the complexity and scope of the business challenges are much greater than for most private sector organizations. To address significant challenges like homelessness, climate change, pandemic response or economic recovery requires a coordination of the legislative, regulatory and policy frameworks across a larger number of stakeholders. The socio-technical complexity for management and decision making is multiplied in this context.
We are seeing those efforts to apply advanced analytics and AI to these large problems are acting as a catalyst to break down the silos within and between government organizations and across jurisdictions. As governments bring together diverse data, technology and domain expertise in governed and open platforms, these ecosystems are driving unique innovation. The opportunity for all stakeholders to become simultaneously more collaborative and autonomous will drive better outcomes for communities.